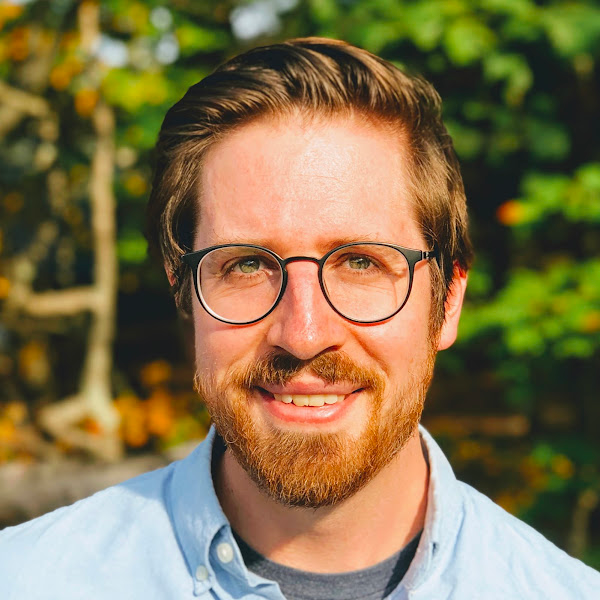
Scott Wisdom
I am a researcher in Google AI Perception in Cambridge, MA working on speech, audio, and audio-visual machine perception, with a focus on audio source separation.
Research Areas
Authored Publications
Sort By
TokenSplit: Using Discrete Speech Representations for Direct, Refined, and Transcript-Conditioned Speech Separation and Recognition
Xuankai Chang
Neil Zeghidour
Interspeech 2023
Preview abstract
We present TokenSplit, a speech separation model that acts on discrete token sequences. The model is trained on multiple tasks simultaneously: separate and transcribe each speech source, and generate speech from text. The model operates on transcripts and audio token sequences and achieves multiple tasks through masking of inputs. The model is a sequence-to-sequence encoder-decoder model that uses the Transformer architecture. We also present a "refinement" version of the model that predicts enhanced audio tokens from the audio tokens of speech separated by a conventional separation model. Using both objective metrics and subjective MUSHRA listening tests, we show that our model achieves excellent performance in terms of separation, both with or without transcript conditioning. We also measure the automatic speech recognition (ASR) performance and provide audio samples of speech synthesis to demonstrate the additional utility of our model.
View details
Don’t Listen to What You Can’t See: The Importance of Negative Examples for Audio-Visual On-Screen Sound Separation
ECCV 2022 Workshop on AV4D: Visual Learning of Sounds in Spaces
Preview abstract
For the task of audio-visual on-screen sound separation, we illustrate the importance of using evaluation sets that includes not only positive examples (videos with on-screen sounds), but also negative examples (videos that only contain off-screen sounds). Given an evaluation set that includes such examples, we provide metrics and a calibration procedure to allow fair comparison of different models with a single metric, which is analogous to calibrating binary classifiers to achieve a desired false alarm rate. In addition, we propose a method of probing on-screen sound separation models by masking objects in input video frames. Using this method, we probe the sensitivity of our recently-proposed AudioScopeV2 model, and discover that its robustness to removing on-screen sound objects is improved by providing supervised examples in training.
View details
Preview abstract
Understanding speech in the presence of noise with hearing aids can be challenging. Here we describe our entry, submission E003, to the 2021 Clarity Enhancement Challenge Round1 (CEC1), a machine learning challenge for improving hearing aid processing. We apply and evaluate a deep neural network speech enhancement model with a low-latency recursive least squares (RLS) adaptive beamformer, and a linear equalizer, to improve speech intelligibility in the presence of speech or noise interferers. The enhancement network is trained only on the CEC1 data, and all processing obeys the 5 ms latency requirement. We quantify the improvement using the CEC1 provided hearing loss model and Modified Binaural Short-Time Objective Intelligibility (MBSTOI) score (ranging from 0 to 1, higher being better). On the CEC1 test set, we achieve a mean of 0.644 and median of 0.652 compared to the 0.310 mean and 0.314 median for the baseline. In the CEC1 subjective listener intelligibility assessment, for scenes with noise interferers, we achieve the second highest improvement in intelligibility from 33.2% to 85.5%, but for speech interferers, we see more mixed results, potentially from listener confusion.
View details
Preview abstract
This paper addresses the problem of species classification in bird song recordings. The massive amount of available field recordings of birds presents an opportunity to use machine learning to automatically track bird populations. However, it also poses a problem: such field recordings typically contain significant environmental noise and overlapping vocalizations that interfere with classification. The widely available training datasets for species identification also typically leave background species unlabeled. This leads classifiers to ignore vocalizations with a low signal-to-noise ratio. However, recent advances in unsupervised sound separation, such as mixture invariant training (MixIT), enable high quality separation of bird songs to be learned from such noisy recordings. In this paper, we demonstrate improved separation quality when training a MixIT model specifically for birdsong data, outperforming a general audio separation model by over 5 dB in SI-SNR improvement of reconstructed mixtures. We also demonstrate precision improvements with a downstream multi-species bird classifier across three independent datasets. The best classifier performance is achieved by taking the maximum model activations over the separated channels and original audio. Finally, we document additional classifier improvements, including taxonomic classification, augmentation by random low-pass filters, and additional channel normalization.
View details
AudioScopeV2: Audio-Visual Attention Architectures for Calibrated Open-Domain On-Screen Sound Separation
Tal Remez
European Conference on Computer Vision (ECCV)(2022)
Preview abstract
We introduce AudioScopeV2, a state-of-the-art universal audio-visual on-screen sound separation system which is capable of learning to separate sounds and associate them with on-screen objects by looking at in-the-wild videos. We identify several limitations of previous work on audio-visual on-screen sound separation, including the coarse resolution of spatio-temporal attention, poor convergence of the audio separation model, limited variety in training and evaluation data, and failure to account for the trade off between preservation of on-screen sounds and suppression of off-screen sounds. We provide solutions to all of these issues. Our proposed cross-modal and self-attention network architectures capture audio-visual dependencies at a finer resolution over time, and we also propose efficient separable variants that are capable of scaling to longer videos without sacrificing much performance. We also find that pre-training the separation model only on audio greatly improves results. For training and evaluation, we collected new human annotations of onscreen sounds from a large database of in-the-wild videos (YFCC100M). This new dataset is more diverse and challenging. Finally, we propose a calibration procedure that allows exact tuning of on-screen reconstruction versus off-screen suppression, which greatly simplifies comparing performance between models with different operating points. Overall, our experimental results show marked improvements in on-screen separation performance under much more general conditions than previous methods with minimal additional computational complexity.
View details
Preview abstract
The recently-proposed mixture invariant training (MixIT) is an unsupervised method for training single-channel sound separation models in the sense that it does not require ground-truth isolated reference sources. In this paper, we investigate using MixIT to adapt a separation model on real far-field overlapping reverberant and noisy speech data from the AMI Corpus. The models are tested on real AMI recordings containing overlapping speech, and are evaluated subjectively by human listeners. To objectively evaluate our models, we also devise a synthetic AMI test set. For human evaluations on real recordings, we also propose a modification of the standard MUSHRA protocol to handle imperfect reference signals, which we call MUSHIRA. Holding network architectures constant, we find that a fine-tuned semi-supervised model yields the largest SI-SNR improvement, PESQ scores, and human listening ratings across synthetic and real datasets, outperforming unadapted generalist models trained on orders of magnitude more data. Our results show that unsupervised learning through MixIT enables model adaptation on real-world unlabeled spontaneous speech recordings.
View details
DF-Conformer: Integrated architecture of Conv-TasNet and Conformer using linear complexity self-attention for speech enhancement
Lion Jones
Proc. IEEE Workshop Appl. Signal Process. Audio Acoust. (WASPAA)(2021)
Preview abstract
Combinations of a trainable filterbank and a mask prediction network is a strong framework in single-channel speech enhancement (SE). Since the denoising performance and computational efficiency are mainly affected by the structure of the mask prediction network, we aim to improve this network. In this study, by focusing on a similarity between the structure of Conv-TasNet and Conformer, we integrate the Conformer into SE as a mask prediction network to benefit its powerful sequential modeling ability. To improve the computational complexity and local sequential modeling, we extend the Conformer using linear complexity attention and stacked 1-D dilated depthwise convolution layers. Experimental results show that (i) the use of linear complexity attention avoids high computational complexity, and (ii) our model achieves higher scale-invariant signal-to-noise ratio than the improved time-dilated convolution network (TDCN++), an extended version of Conv-TasNet.
View details
What's All the FUSS About Free Universal Sound Separation Data?
Romain Serizel
Nicolas Turpault
Eduardo Fonseca
Justin Salamon
Prem Seetharaman
ICASSP 2021
Preview abstract
We introduce the Free Universal Sound Separation (FUSS) dataset, a new corpus for experiments in separating mixtures of an unknown number of sounds from an open domain of sound types. The dataset consists of 23 hours of single-source audio data drawn from 357 classes, which are used to create mixtures of one to four sources. To simulate reverberation, an acoustic room simulator is used to generate impulse responses of box shaped rooms with frequency-dependent reflective walls. Additional open-source data augmentation tools are also provided to produce new mixtures with different combinations of sources and room simulations. Finally, we introduce an open-source baseline separation model, based on an improved time-domain convolutional network (TDCN++), that can separate a variable number of sources in a mixture. This model achieves 9.8 dB of scale-invariant signal-to-noise ratio improvement (SI-SNRi) on mixtures with two to four sources, while reconstructing single-source inputs with 35.5 dB absolute SI-SNR. We hope this dataset will lower the barrier to new research and allow for fast iteration and application of novel techniques from other machine learning domains to the sound separation challenge.
View details
Sparse, Efficient, and Semantic MixIT: Taming In-the-Wild Unsupervised Sound Separation
Proceedings of the IEEE Workshop on Applications of Signal Processing to Audio and Acoustics (WASPAA)(2021)
Preview abstract
Supervised neural network training has led to significant progress on single-channel sound separation. This approach relies on ground truth isolated sources, which precludes scaling to widely available mixture data and limits progress on open-domain tasks. The recent mixture invariant training (MixIT) method enables training on in-the-wild data; however, it suffers from two outstanding problems. First, it produces models which tend to over-separate, producing more output sources than are present in the input. Second, the exponential computational complexity of the MixIT loss limits the number of feasible output sources. In this paper we address both issues. To combat over-separation we introduce new losses: sparsity losses that favor fewer output sources and a covariance loss that discourages correlated outputs. We also experiment with a semantic classification loss by predicting weak class labels for each mixture. To handle larger numbers of sources, we introduce an efficient approximation using a fast least-squares solution, projected onto the MixIT constraint set. Our experiments show that the proposed losses curtail over-separation and improve overall performance. The best performance is achieved using larger numbers of output sources, enabled by our efficient MixIT loss, combined with sparsity losses to prevent over-separation. On the FUSS test set, we achieve over 13 dB in multi-source SI-SNR improvement, while boosting single-source reconstruction SI-SNR by over 17 dB.
View details
Sequential Multi-Frame Neural Beamforming for Speech Separation and Enhancement
Zhong-Qiu Wang
Desh Raj
Shinji Watanabe
Zhuo Chen
IEEE SLT 2021
Preview abstract
This work introduces sequential neural beamforming, which alternates between neural network based spectral separation and beamforming based spatial separation. Our neural networks for separation use an advanced convolutional architecture trained with a novel stabilized signal-to-noise ratio loss function. For beamforming, we explore multiple ways of computing time-varying covariance matrices, including factorizing the spatial covariance into a time-varying amplitude component and a time-invariant spatial component, as well as using block-based techniques. In addition, we introduce a multi-frame beamforming method which improves the results significantly by adding contextual frames to the beamforming formulations. We extensively evaluate and analyze the effects of window size, block size, and multi-frame context size for these methods. Our best method utilizes a sequence of three neural separation and multiframe time-invariant spatial beamforming stages, and demonstrates an average improvement of 2.75 dB in scale-invariant signal-to-noise ratio and 14.2% absolute reduction in a comparative speech recognition metric across four challenging reverberant speech enhancement and separation tasks. We also use our three-speaker separation model to separate real recordings in the LibriCSS evaluation set into non-overlapping tracks, and achieve a better word error rate as compared to a baseline mask based beamformer.
View details