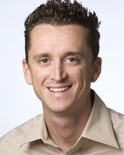
Luca Bertelli
Luca was born in a small town in northern Italy. He received the D.Ing. degree (summa cum laude) in electronic engineering from the University of Modena, Italy, in 2003, and the M.S. and Ph.D. degrees in electrical and computer engineering from the University of California, Santa Barbara, in 2005 and 2009, respectively. During the summer of 2008, he was an intern here at Google working on salient object detection within the computer vision research group. He then re-joined Google in the summer of 2010 through the Like.com acquisition, and he has been working on the Boutiques.com computer-vision related aspects since.
Personal home page
Research Areas
Authored Publications
Google Publications
Other Publications
Sort By
Preview abstract
Large Language Models (LLMs) have shown impressive results on a variety of text understanding tasks. Search queries though pose a unique challenge, given their short-length and lack of nuance or context. Complicated feature engineering efforts do not always lead to downstream improvements as their performance benefits may be offset by increased complexity of knowledge distillation. Thus, in this paper we make the following contributions: (1) We demonstrate that Retrieval Augmentation of queries provides LLMs with valuable additional context enabling improved understanding. While Retrieval Augmentation typically increases latency of LMs (thus hurting distillation efficacy), (2) we provide a practical and effective way of distilling Retrieval Augmentation LLMs. Specifically, we use a novel two-stage distillation approach that allows us to carry over the gains of retrieval augmentation, without suffering the increased compute typically associated with it. (3) We demonstrate the benefits of the proposed approach on a billion-scale, real-world query understanding system resulting in an X\% improvement. Via extensive experiments, including on public benchmarks, we believe this work offers a recipe for practical use of retrieval-augmented query understanding.
View details
Kernelized Structural SVM Learning for Supervised Object Segmentation
Tianli Yu
Diem Vu
Burak Gokturk
Proceedings of IEEE Conference on Computer Vision and Pattern Recognition 2011
Preview abstract
Object segmentation needs to be driven by top-down knowledge to produce semantically meaningful results. In this paper, we propose a supervised segmentation approach that tightly integrates object-level top down information with low-level image cues. The information from the two levels is fused under a kernelized structural SVM learning framework. We defined a novel nonlinear kernel for comparing two image-segmentation masks. This kernel combines four different kernels: the object similarity kernel, the object shape kernel, the per-image color distribution kernel, and the global color distribution kernel. Our experiments show that the structured SVM algorithm finds bad segmentations of the training examples given the current scoring function and punishes these bad segmentations to lower scores than the example (good) segmentations. The result is a segmentation algorithm that not only knows what good segmentations are, but also learns potential segmentation mistakes and tries to avoid them. Our proposed approach can obtain comparable performance to other state-of-the-art top-down driven segmentation approaches yet is flexible enough to be applied to widely different domains.
View details
No Results Found