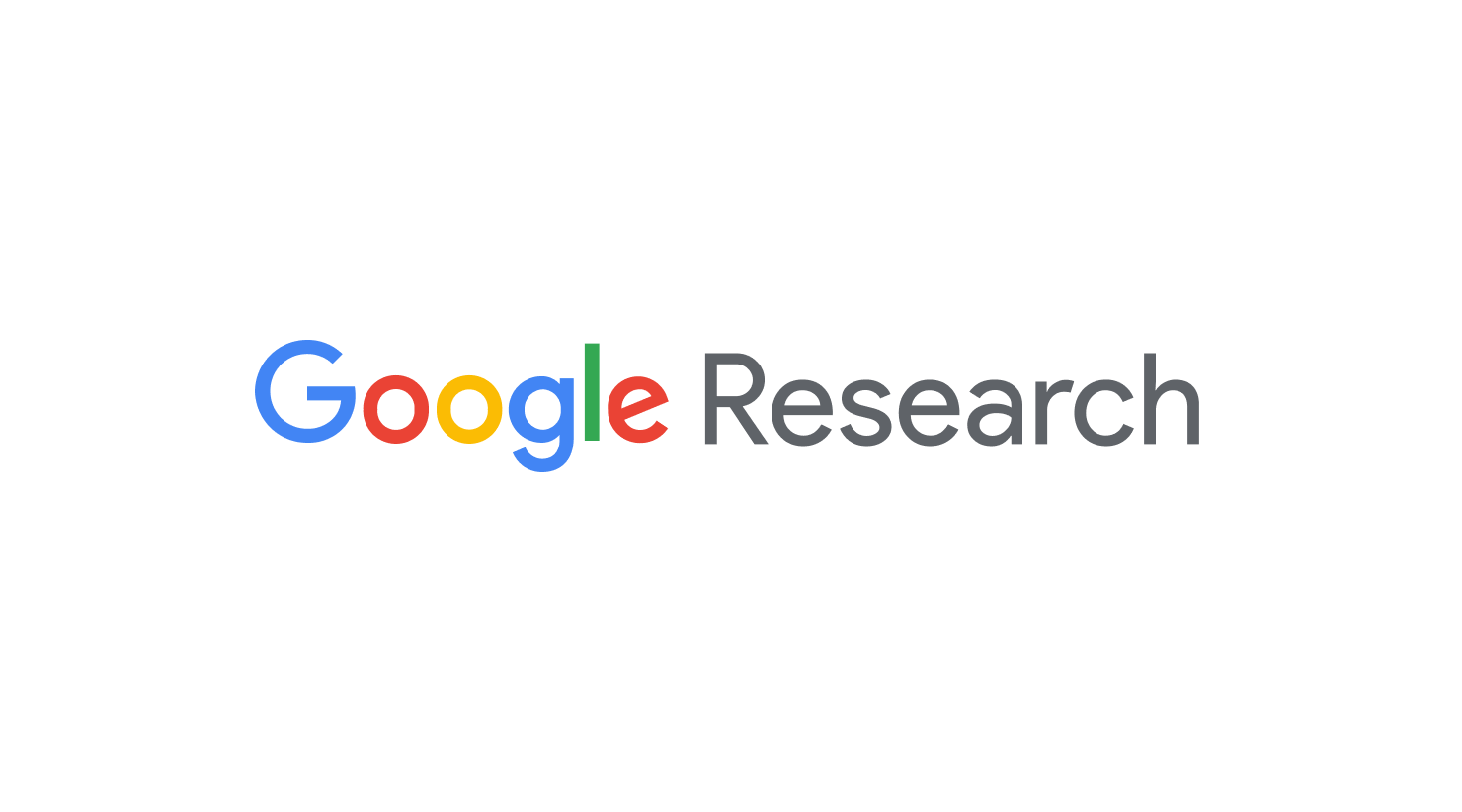
Gamification for Improved Search Ranking for YouTube Topics
March 19, 2012
Posted by Charles DuHadway and Sanketh Shetty, Google Research
In earlier posts we discussed automatic ways to find the most talented emerging singers and the funniest videos using the YouTube Slam experiment. We created five “house” slams -- music, dance, comedy, bizarre, and cute -- which produce a weekly leaderboard not just of videos but also of YouTubers who are great at predicting what the masses will like. For example, last week’s cute slam winning video claims to be the cutest kitten in the world, beating out four other kittens, two puppies, three toddlers and an amazing duck who feeds the fish. With a whopping 620 slam points, YouTube user emoatali99 was our best connoisseur of cute this week. On the music side, it is no surprise that many of music slam’s top 10 videos were Adele covers. A Whitney Houston cover came out at the top this week, and music slam’s resident expert on talent had more than a thousand slam points. Well done! Check out the rest of the leaderboards for cute slam and music slam.
Can slam-style game mechanics incentivize our users to help improve the ranking of videos -- not just for these five house slams -- but for millions of other search queries and topics on YouTube? Gamification has previously been used to incentivize users to participate in non-game tasks such as image labeling and music tagging. How many votes and voters would we need for slam to do better than the existing ranking algorithm for topic search on YouTube?
As an experiment, we created new slams for a small number of YouTube topics (such as Latte Art Slam and Speed Painting Slam) using existing top 20 videos for these topics as the candidate pool. As we accumulated user votes, we evaluated the resulting YouTube Slam leaderboard for that topic vs the existing ranking on youtube.com/topics (baseline). Note that both the slam leaderboard and the baseline had the same set of videos, just in a different order.
What did we discover? It was no surprise that slam ranking performance had a high variance in the beginning and gradually improved as votes accumulated. We are happy to report that four of five topic slams converged within 1000 votes with a better leaderboard ranking than the existing YouTube topic search. In spite of small number of voters, Slam achieves better ranking partly because of gamification incentives and partly because it is based on machine learning, using:
- Preference judgement over a pair, not absolute judgement on a single video, and,
- Active solicitation of user opinion as opposed to passive observation. Due to what is called a “cold start” problem in data modeling, conventional (passive observation) techniques don’t work well on new items with little prior information. For any given topic, Slam’s improvement over the baseline in ranking of the “recent 20” set of videos was in fact better than the improvement in ranking of the “top 20” set.
Demographics and interests of the voters do affect slam leaderboard ranking, especially when the voter pool is small. An example is a Romantic Proposals Slam we featured on Valentine’s day last month. Men thought this proposal during a Kansas City Royals game was the most romantic, although this one where the man pretends to fall off a building came close. On the other hand, women rated this meme proposal in a restaurant as the best, followed by this movie theater proposal.
Encouraged by these results, we will soon be exploring slams for a few thousand topics to evaluate the utility of gamification techniques to YouTube topic search. Here are some of them: Chocolate Brownie, Paper Plane, Bush Flying, Stealth Technology, Stencil Graffiti, Yosemite National Park, and Stealth Technology.
Have fun slamming!