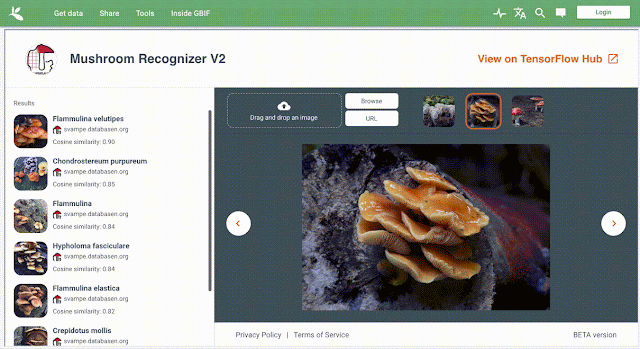
A New Workflow for Collaborative Machine Learning Research in Biodiversity
October 25, 2019
Posted by Serge Belongie, Visiting Faculty and Hartwig Adam, Engineering Director, Google Research
The promise of machine learning (ML) for species identification is coming to fruition, revealing its transformative potential in biodiversity research. International workshops such as FGVC and LifeCLEF feature competitions to develop top performing classification algorithms for everything from wildlife camera trap images to pressed flower specimens on herbarium sheets. The encouraging results that have emerged from these competitions inspired us to expand the availability of biodiversity datasets and ML models from workshop-scale to global-scale.
Bringing powerful ML algorithms to the communities that need them requires more than the traditional “big data + big compute” equation. Institutions ranging from natural history museums to citizen science groups take great care to collect and annotate datasets, and the data they share have enabled numerous scientific research publications. But central to the tradition of scholarly research are the conventions of citation and attribution, and it follows that as ML extends its reach into the life sciences, it should bring with it appropriate counterparts to those conventions. More broadly, there is a growing awareness of the importance of ethics, fairness, and transparency within the ML community. As institutions develop and deploy applications of ML at scale, it is critical that they be designed with these considerations in mind.
This week at Biodiversity Next, in collaboration with the Global Biodiversity Information Facility (GBIF), iNaturalist, and Visipedia, we are announcing a new workflow for biodiversity research institutions who would like to make use of ML. With its billion+ species occurrence count contributed by thousands of institutions around the globe, GBIF is playing a vital role in enabling this workflow, whether in terms of data aggregation, collaboration across teams, or standardizing citation practices. In the short term, the most important role relates to an emerging cultural shift in accepted practices for the use of mediated data for training of ML models. In the process of data mediation, GBIF helps ensure that training datasets for ML follow standardized licensing terms, use compatible taxonomies and data formats, and provide fair and sufficient data coverage for the ML task at hand by potentially sampling from multiple source datasets.
This new workflow comprises the following two components:
- To assist in developing and refining machine vision models, GBIF will package datasets, taking care to ensure license and citation practice are respected. The training datasets will be issued a Digital Object Identifier (DOI), and will be linked through the DOI citation graph.
- To assist application developers, Google and Visipedia will train and publish publicly accessible models with documentation on TensorFlow Hub. These models can then, in turn, be deployed in biodiversity research and citizen science efforts.
As an illustration of the above workflow, we present an example of fungi recognition. The dataset in this case is curated by the Danish Mycological Society, and formatted, packaged, and shared by GBIF. The dataset provenance, model architecture, license information, and more can be found on the TF Hub model page, along with a live, interactive demonstration of the model that can run on user-supplied images.
![]() |
Illustration of live, interactive Mushroom Recognizer, powered by a publicly available model trained on a fungi dataset provided by the Danish Mycological Society. |
For more information about this initiative, please visit the project page at GBIF. We look forward to engaging with institutions around the globe to enable new and innovative uses of ML for biodiversity.
Acknowledgements
We’d like to thank our collaborators at GBIF, iNaturalist, and Visipedia for working together to develop this workflow. At Google we would like to thank Christine Kaeser-Chen, Chenyang Zhang, Yulong Liu, Kiat Chuan Tan, Christy Cui, Arvi Gjoka, Denis Brulé, Cédric Deltheil, Clément Beauseigneur, Grace Chu, Andrew Howard, Sara Beery, and Katherine Chou.