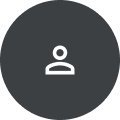
Sai Meher Karthik Duddu
Research Areas
Authored Publications
Google Publications
Other Publications
Sort By
Rethinking the Role of Token Retrieval in Multi-Vector Retrieval
Zhuyun Dai
Tao Lei
Iftekhar Naim
Ming-Wei Chang
Vincent Zhao
Thirty-seventh Conference on Neural Information Processing Systems (NeurIPS 2023)
Preview abstract
Multi-vector retrieval models such as ColBERT [Khattab and Zaharia, 2020] allow token-level interactions between queries and documents, and hence achieve state of the art on many information retrieval benchmarks. However, their non-linear scoring functions are computationally expensive, necessitating a two-stage process for inference: initial candidate retrieval via token retrieval and subsequent refinement stage which re-ranks candidates using the scoring function. Prior training algorithms mainly focus on the re-ranking stage, under-estimating the importance of the token retrieval stage. In this paper, we rethink the role of token retrieval for multi-vector retrieval models and presentXTR, ConteXtualized TokenRetriever. XTR introduces a simple, yet novel, objective function to encourage better token retrieval, which drastically reduce the mismatch between the training objective and the inference procedure. Unexpectedly, our studies have demonstrated that when the token retrieval stage is improved, the refinement stage can be reduced and approximated. Based on this observation, XTR includes a fast refinement algorithm that can re-rank the candidates 4,000× cheaper compared to the refinement stage of ColBERT. On the popular BEIR benchmark [Thakur et al., 2021], XTR advances the state-of-the-art by 3.3 points, achieving 53.2 nDCG@10. Detailed analysis is conducted to confirm that the success of XTR indeed come from better recall of the token-level retrieval stage.
View details
No Results Found