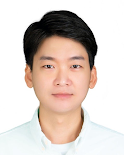
Jinhyuk Lee
Authored Publications
Google Publications
Other Publications
Sort By
Preview abstract
Recent developments of dense retrieval rely on quality representations of queries and contexts from pre-trained query and context encoders. In this paper, we introduce TOUR (Test-Time Optimization of Query Representations), which further optimizes instance-level query representations guided by signals from test-time retrieval results. We leverage a cross-encoder re-ranker to provide fine-grained pseudo labels over retrieval results and iteratively optimize query representations with gradient descent. Our theoretical analysis reveals that TOUR can be viewed as a generalization of the classical Rocchio algorithm for pseudo relevance feedback, and we present two variants that leverage pseudo-labels as hard binary or soft continuous labels. We first apply TOUR on phrase retrieval with our proposed phrase re-ranker, and also evaluate its effectiveness on passage retrieval with an off-the-shelf re-ranker. TOUR greatly improves end-to-end open-domain question answering accuracy, as well as passage retrieval performance. TOUR also consistently improves direct re-ranking by up to 2.0% while running 1.3-2.4x faster with an efficient implementation.
View details
MoQA: Benchmarking Multi-Type Open-Domain Question Answering
Howard Yen
Tianyu Gao
Danqi Chen
Proceedings of the Third DialDoc Workshop on Document-grounded Dialogue and Conversational Question Answering, Association for Computational Linguistics (2023), 8–29
Preview abstract
Existing open-domain question answering research mainly focuses on questions that can be answered in a few words.
However, information-seeking questions often require different formats of answers depending on the nature of questions, e.g., ``Why is there a maple leaf on the Canadian flag?''
In this paper, we present a new task, MOQA, which requires building QA models that can provide short, medium, long, and yes/no answers to open-domain questions simultaneously.
We expand the Natural Questions dataset into the open-domain setting by keeping all types of questions and show that existing systems cannot generalize to these new types.
We adapt state-of-the-art open-domain QA models---based on retriever-reader and phrase retrieval models---to tackle this task.
Results and analyses of our multi-type QA models reveal the unique challenges of the task, calling for versatile QA models in the future.
View details
Rethinking the Role of Token Retrieval in Multi-Vector Retrieval
Zhuyun Dai
Tao Lei
Iftekhar Naim
Ming-Wei Chang
Vincent Zhao
Thirty-seventh Conference on Neural Information Processing Systems (NeurIPS 2023)
Preview abstract
Multi-vector retrieval models such as ColBERT [Khattab and Zaharia, 2020] allow token-level interactions between queries and documents, and hence achieve state of the art on many information retrieval benchmarks. However, their non-linear scoring functions are computationally expensive, necessitating a two-stage process for inference: initial candidate retrieval via token retrieval and subsequent refinement stage which re-ranks candidates using the scoring function. Prior training algorithms mainly focus on the re-ranking stage, under-estimating the importance of the token retrieval stage. In this paper, we rethink the role of token retrieval for multi-vector retrieval models and presentXTR, ConteXtualized TokenRetriever. XTR introduces a simple, yet novel, objective function to encourage better token retrieval, which drastically reduce the mismatch between the training objective and the inference procedure. Unexpectedly, our studies have demonstrated that when the token retrieval stage is improved, the refinement stage can be reduced and approximated. Based on this observation, XTR includes a fast refinement algorithm that can re-rank the candidates 4,000× cheaper compared to the refinement stage of ColBERT. On the popular BEIR benchmark [Thakur et al., 2021], XTR advances the state-of-the-art by 3.3 points, achieving 53.2 nDCG@10. Detailed analysis is conducted to confirm that the success of XTR indeed come from better recall of the token-level retrieval stage.
View details
No Results Found