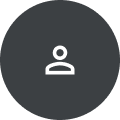
Yotaro Kubo
Research Areas
Authored Publications
Sort By
Preview abstract
End-to-end speech recognition is a promising technology for enabling compact automatic speech recognition (ASR) systems since it can unify the acoustic and language model into a single neural network.
However, as a drawback, training of end-to-end speech recognizers always requires transcribed utterances.
Since end-to-end models are also known to be severely data hungry, this constraint is crucial especially because obtaining transcribed utterances is costly and can possibly be impractical or impossible.
This paper proposes a method for alleviating this issue by transferring knowledge from a language model neural network that can be pretrained with text-only data.
Specifically, this paper attempts to transfer semantic knowledge acquired in embedding vectors of large-scale language models.
Since embedding vectors can be assumed as implicit representations of linguistic information such as part-of-speech, intent, and so on, those are also expected to be useful modeling cues for ASR decoders.
This paper extends two types of ASR decoders, attention-based decoders and neural transducers, by modifying training loss functions to include embedding prediction terms.
The proposed systems were shown to be effective for error rate reduction without incurring extra computational costs in the decoding phase.
View details
A Comparative Study on Neural Architectures and Training Methods for Japanese Speech Recognition
Lion Jones
Interspeech 2021 (2021) (to appear)
Preview abstract
End-to-end (E2E) modeling is advantageous for automatic speech recognition (ASR) especially for Japanese since word-based tokenization of Japanese is not trivial, and E2E modeling is able to model character sequences directly. This paper focuses on the latest E2E modeling techniques, and investigates their performances on character-based Japanese ASR by conducting comparative experiments. The results are analyzed and discussed in order to understand the relative advantages of long short-term memory (LSTM), and Conformer models in combination with connectionist temporal classification, transducer, and attention-based loss functions. Furthermore, the paper investigates on effectivity of the recent training techniques such as data augmentation (SpecAugment), variational noise injection, and exponential moving average. The best configuration found in the paper achieved the state-of-the-art character error rates of 4.1%, 3.2%, and 3.5% for Corpus of Spontaneous Japanese (CSJ) eval1, eval2, and eval3 tasks, respectively. The system is also shown to be computationally efficient thanks to the efficiency of Conformer transducers.
View details
Preview abstract
This paper proposes methods to improve a commonly used end-to-end speech recognition model, Listen-Attend-Spell (LAS).
The methods we propose use multi-task learning to improve generalization of the model by leveraging information from multiple labels.
The focus in this paper is on multi-task models for simultaneous signal-to-grapheme and signal-to-phoneme conversions while sharing the encoder parameters.
Since phonemes are designed to be a precise description of the linguistic aspects of the speech signal, using phoneme recognition as an auxiliary task can help guiding the early stages of training to be more stable.
In addition to conventional multi-task learning, we obtain further improvements by introducing a method that can exploit dependencies between labels in different tasks. Specifically, the dependencies between phonemes and grapheme sequences are considered. In conventional multi-task learning these sequences are assumed to be independent. Instead, in this paper, a joint model is proposed based on ``iterative refinement'' where dependency modeling is achieved by a multi-pass strategy.
The proposed method is evaluated on a 28000h corpus of Japanese speech data. Performance of a conventional multi-task approach is contrasted with that of the joint model with iterative refinement.
View details