Publications
Our teams aspire to make discoveries that impact everyone, and core to our approach is sharing our research and tools to fuel progress in the field.
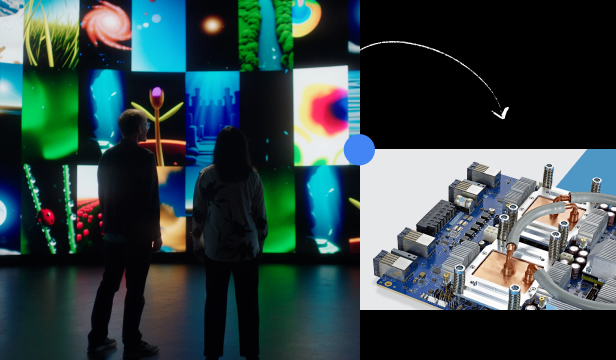
Publications
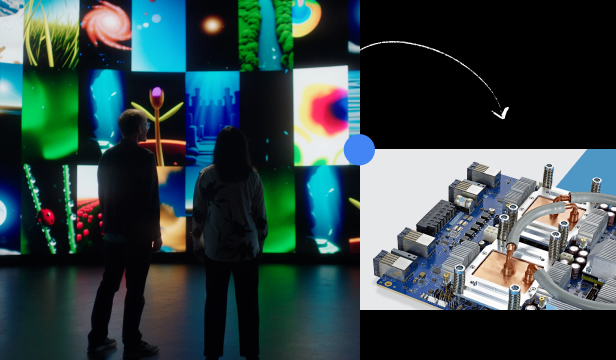
Sort By
1 - 15 of 227 publications
Generative AI in Creative Practice: ML-Artist Folk Theories of T2I Use, Harm, and Harm-Reduction
Shalaleh Rismani
Proceedings of the CHI Conference on Human Factors in Computing Systems (CHI '24), Association for Computing Machinery(2024), pp. 1-17 (to appear)
Preview abstract
Understanding how communities experience algorithms is necessary to mitigate potential harmful impacts. This paper presents folk theories of text-to-image (T2I) models to enrich understanding of how artist communities experience creative machine learning (ML) systems. This research draws on data collected from a workshop with 15 artists from 10 countries who incorporate T2I models in their creative practice. Through reflexive thematic analysis of workshop data, we highlight theorization of T2I use, harm, and harm-reduction. Folk theories of use envision T2I models as an artistic medium, a mundane tool, and locate true creativity as rising above model affordances. Theories of harm articulate T2I models as harmed by engineering efforts to eliminate glitches and product policy efforts to limit functionality. Theories of harm-reduction orient towards protecting T2I models for creative practice through transparency and distributed governance. We examine how these theories relate, and conclude by discussing how folk theorization informs responsible AI efforts.
View details
Take it, Leave it, or Fix it: Measuring Productivity and Trust in Human-AI Collaboration
29th International Conference on Intelligent User Interfaces (IUI ’24), ACM, New York, NY, USA(2024)
Preview abstract
Although recent developments in generative AI have greatly enhanced the capabilities of conversational agents such as Google's Bard or OpenAI's ChatGPT, it's unclear whether the usage of these agents aids users across various contexts. To better understand how access to conversational AI affects productivity and trust, we conducted a mixed-methods, task-based user study, observing 76 software engineers (N=76) as they completed a programming exam with and without access to Bard. Effects on performance, efficiency, satisfaction, and trust vary depending on user expertise, question type (open-ended "solve" questions vs. definitive "search" questions), and measurement type (demonstrated vs. self-reported). Our findings include evidence of automation complacency, increased reliance on the AI over the course of the task, and increased performance for novices on “solve”-type questions when using the AI. We discuss common behaviors, design recommendations, and impact considerations to improve collaborations with conversational AI.
View details
Generative models improve fairness of medical classifiers under distribution shifts
Ira Ktena
Olivia Wiles
Isabela Albuquerque
Sylvestre-Alvise Rebuffi
Ryutaro Tanno
Danielle Belgrave
Taylan Cemgil
Nature Medicine(2024)
Preview abstract
Domain generalization is a ubiquitous challenge for machine learning in healthcare. Model performance in real-world conditions might be lower than expected because of discrepancies between the data encountered during deployment and development. Underrepresentation of some groups or conditions during model development is a common cause of this phenomenon. This challenge is often not readily addressed by targeted data acquisition and ‘labeling’ by expert clinicians, which can be prohibitively expensive or practically impossible because of the rarity of conditions or the available clinical expertise. We hypothesize that advances in generative artificial intelligence can help mitigate this unmet need in a steerable fashion, enriching our training dataset with synthetic examples that address shortfalls of underrepresented conditions or subgroups. We show that diffusion models can automatically learn realistic augmentations from data in a label-efficient manner. We demonstrate that learned augmentations make models more robust and statistically fair in-distribution and out of distribution. To evaluate the generality of our approach, we studied three distinct medical imaging contexts of varying difficulty: (1) histopathology, (2) chest X-ray and (3) dermatology images. Complementing real samples with synthetic ones improved the robustness of models in all three medical tasks and increased fairness by improving the accuracy of clinical diagnosis within underrepresented groups, especially out of distribution.
View details
Automatic Speech Recognition of Conversational Speech in Individuals with Disordered Speech
Bob MacDonald
Rus Heywood
Richard Cave
Katie Seaver
Antoine Desjardins
Jordan Green
Journal of Speech, Language, and Hearing Research(2024) (to appear)
Preview abstract
Purpose: This study examines the effectiveness of automatic speech recognition (ASR) for individuals with speech disorders, addressing the gap in performance between read and conversational ASR. We analyze the factors influencing this disparity and the effect of speech mode-specific training on ASR accuracy.
Method: Recordings of read and conversational speech from 27 individuals with various speech disorders were analyzed using both (1) one speaker-independent ASR system trained and optimized for typical speech and (2) multiple ASR models that were personalized to the speech of the participants with disordered speech. Word Error Rates (WERs) were calculated for each speech mode, read vs conversational, and subject. Linear mixed-effect models were used to assess the impact of speech mode and disorder severity on ASR accuracy. We investigated nine variables, classified as technical, linguistic, or speech impairment factors, for their potential influence on the performance gap.
Results: We found a significant performance gap between read and conversational speech in both personalized and unadapted ASR models. Speech impairment severity notably impacted recognition accuracy in unadapted models for both speech modes and in personalized models for read speech. Linguistic attributes of utterances were the most influential on accuracy, though atypical speech characteristics also played a role. Including conversational speech samples in model training notably improved recognition accuracy.
Conclusions: We observed a significant performance gap in ASR accuracy between read and conversational speech for individuals with speech disorders. This gap was largely due to the linguistic complexity and unique characteristics of speech disorders in conversational speech. Training personalized ASR models using conversational speech significantly improved recognition accuracy, demonstrating the importance of domain-specific training and highlighting the need for further research into ASR systems capable of handling disordered conversational speech effectively.
View details
Large Language Models as a Proxy For Human Evaluation in Assessing the Comprehensibility of Disordered Speech Transcription
Subhashini Venugopalan
Richard Cave
Katie Seaver
Jordan Green
Rus Heywood
Proceedings of ICASSP, IEEE(2024)
Preview abstract
Automatic Speech Recognition (ASR) systems, despite significant advances in recent years, still have much room for improvement particularly in the recognition of disordered speech. Even so, erroneous transcripts from ASR models can help people with disordered speech be better understood, especially if the transcription doesn’t significantly change the intended meaning. Evaluating the efficacy of ASR for this use case requires a methodology for measuring the impact of transcription errors on the intended meaning and comprehensibility. Human evaluation is the gold standard for this, but it can be laborious, slow, and expensive. In this work, we tune and evaluate large language models for this task and find them to be a much better proxy for human evaluators than other metrics commonly used. We further present a case-study using the presented approach to assess the quality of personalized ASR models to make model deployment decisions and correctly set user expectations for model quality as part of our trusted tester program.
View details
Preview abstract
Language models still struggle on moral reasoning, despite their impressive performance in many other tasks. In particular, the Moral Scenarios task in MMLU (Multi-task Language Understanding) is among the worst performing tasks for many language models, including GPT-3. In this work, we propose a new prompting framework, Thought Experiments, to teach language models to do better moral reasoning using counterfactuals. Experiment results show that our framework elicits counterfactual questions and answers from the model, which in turn helps improve the accuracy on Moral Scenarios task by 9-16% compared to other zero-shot baselines. Interestingly, unlike math reasoning tasks, zero-shot Chain-of-Thought (CoT) reasoning doesn't work out of the box, and even reduces accuracy by around 4% compared to direct zero-shot. We further observed that with minimal human supervision in the form of 5 few-shot examples, the accuracy of the task can be improved to as much as 80%.
View details
AI Consent Futures: A Case Study on Voice Data Collection with Clinicians
Lauren Wilcox
Robin Brewer
Fernando Diaz
ACM CSCW(2023) (to appear)
Preview abstract
As new forms of data capture emerge to power new AI applications, questions abound about the ethical implications of these data collection practices. In this paper, we present clinicians' perspectives on the prospective benefits and harms of voice data collection during health consultations. Such data collection is being proposed as a means to power models to assist clinicians with medical data entry, administrative tasks, and consultation analysis. Yet, clinicians' attitudes and concerns are largely absent from the AI narratives surrounding these use cases, and the academic literature investigating them. Our qualitative interview study used the concept of an informed consent process as a type of design fiction, to support elicitation of clinicians' perspectives on voice data collection and use associated with a fictional, near-term AI assistant. Through reflexive thematic analysis of in-depth sessions with physicians, we distilled eight classes of potential risks that clinicians are concerned about, including workflow disruptions, self-censorship, and errors that could impact patient eligibility for services. We conclude with an in-depth discussion of these prospective risks, reflect on the use of the speculative processes that illuminated them, and reconsider evaluation criteria for AI-assisted clinical documentation technologies in light of our findings.
View details
Public Health Calls for/with AI: An Ethnographic Perspective
Azra Ismail
Neha Kumar
Neha Madhiwalla
ACM Conference On Computer-Supported Cooperative Work And Social Computing(2023)
Preview abstract
Artificial Intelligence (AI) based technologies are increasingly being integrated into public sector programs to help with decision-support and effective distribution of constrained resources. The field of Computer Supported Cooperative Work (CSCW) has begun to examine how the resultant sociotechnical systems may be designed appropriately when targeting underserved populations. We present an ethnographic study of a largescale real-world integration of an AI system for resource allocation in a call-based maternal and child health
program in India. Our findings uncover complexities around determining who benefits from the intervention, how the human-AI collaboration is managed, when intervention must take place in alignment with various priorities, and why the AI is sought, for what purpose. Our paper offers takeaways for human-centered AI integration in public health, drawing attention to the work done by the AI as actor, the work of configuring the human-AI partnership with multiple diverse stakeholders, and the work of aligning program goals for design and implementation through continual dialogue across stakeholders.
View details
Preview abstract
Along with the recent advances in large language modeling, there is growing concern that language technologies may reflect, propagate, and amplify various social stereotypes about groups of people. Publicly available stereotype benchmarks play a crucial role in detecting and mitigating this issue in language technologies to prevent both representational and allocational harms in downstream applications. However, existing stereotype benchmarks are limited in their size and coverage, largely restricted to stereotypes prevalent in the Western society. This is especially problematic as language technologies are gaining hold across the globe. To address this gap, we present SeeGULL, a broad-coverage stereotype dataset, expanding the coverage by utilizing the generative capabilities of large language models such as PaLM and GPT-3, and leveraging a globally diverse rater pool to validate prevalence of those stereotypes in society. SeeGULL is an order of magnitude larger in terms of size, and contains stereotypes for 179 identity groups spanning 6 continents, 8 different regions, 178 countries, 50 US states, and 31 Indian states and union territories. We also get fine-grained offensiveness scores for different stereotypes and demonstrate how stereotype perceptions for the same identity group differs across in-region vs out-region annotators.
View details
Infrastructuring Care: How Trans and Non-Binary People Meet Health and Well-Being Needs through Technology
Lauren Wilcox
Rajesh Veeraraghavan
Oliver Haimson
Gabi Erickson
Michael Turken
Beka Gulotta
ACM Conference on Human Factors in Computing Systems (ACM CHI) 2023, Association for Computing Machinery, ACM(2023)
Preview abstract
We present a cross-cultural diary study with 64 transgender (trans) and non-binary (TGNB) adults in Mexico, the U.S., and India, to understand experiences keeping track of and managing aspects of personal health and well-being. Based on a reflexive thematic analysis of diary data, we highlight sociotechnical interactions that shape how transgender and non-binary people track and manage aspects of their health and well-being. Specifically, we surface the ways in which transgender and non-binary people infrastructure forms of care, by assembling together elements of informal social ecologies, formalized knowledge sources, and self-reflective media. We then examine the forms of precarity that interact with care infrastructure and shape management of health and well-being, including management of gender identity transitions. We discuss the ways in which our findings extend knowledge at the intersection of technology and marginalized health needs, and conclude by arguing for the importance of a research agenda to move toward TGNB-inclusive design.
View details
Contextualizing the Limits of Model & Evaluation Dataset Curation on Semantic Similarity Classification Tasks
EMNLP Generation, Evaluation & Metrics (GEM) Workshop(2023)
Preview abstract
This paper demonstrates how the limitations of pre-trained models and open evaluation datasets factor into assessing the performance of binary semantic similarity classification tasks. As (1) end-user-facing documentation around the curation of these datasets and pre-trained model training regimes is often not easily accessible and (2) given the lower friction and higher demand to quickly deploy such systems in real-world contexts, our study reinforces prior work showing performance disparities across datasets, embedding techniques and distance metrics, while highlighting the importance of understanding how data is collected, curated and analyzed in semantic similarity classification.
View details
AI’s Regimes of Representation: A Community-centered Study of Text-to-Image Models in South Asia
Rida Qadri
Proceedings of the 2023 ACM Conference on Fairness, Accountability, and Transparency, Association for Computing Machinery, 506–517
Preview abstract
This paper presents a community-centered study of cultural limitations of text-to-image (T2I) models in the South Asian context. We theorize these failures using scholarship on dominant media regimes of representations and locate them within participants’ reporting of their existing social marginalizations. We thus show how generative AI can reproduce an outsiders gaze for viewing South Asian cultures, shaped by global and regional power inequities. By centering communities as experts and soliciting their perspectives on T2I limitations, our study adds rich nuance into existing evaluative frameworks and deepens our understanding of the culturally-specific ways AI technologies can fail in non-Western and Global South settings. We distill lessons for responsible development of T2I models, recommending concrete pathways forward that can allow for recognition of structural inequalities.
View details
VLSlice: Interactive Vision-and-Language Slice Discovery
Eric Slyman
Stefan Lee
Proceedings of the IEEE/CVF International Conference on Computer Vision (ICCV)(2023), pp. 15291-15301
Preview abstract
Recent work in vision-and-language demonstrates that large-scale pretraining can learn generalizable models that are efficiently transferable to downstream tasks. While this may improve dataset-scale aggregate metrics, analyzing performance around hand-crafted subgroups targeting specific bias dimensions reveals systemic undesirable behaviors. However, this subgroup analysis is frequently stalled by annotation efforts, which require extensive time and resources to collect the necessary data. Prior art attempts to automatically discover subgroups to circumvent these constraints but typically leverages model behavior on existing task-specific annotations and rapidly degrades on more complex inputs beyond "tabular" data, none of which study vision-and-language models. This paper presents VLSlice, an interactive system enabling user-guided discovery of coherent representation-level subgroups with consistent visiolinguistic behavior, denoted as vision-and-language slices, from unlabeled image sets. We show that VLSlice enables users to quickly generate diverse high-coherency slices in a user study (n=22) and release the tool publicly.
View details
Identifying Sociotechnical Harms of Algorithmic Systems: Scoping a Taxonomy for Harm Reduction
Shalaleh Rismani
Kathryn Henne
AJung Moon
Paul Nicholas
N'Mah Yilla-Akbari
Jess Gallegos
Emilio Garcia
Gurleen Virk
Proceedings of the 2023 AAAI/ACM Conference on AI, Ethics, and Society, Association for Computing Machinery, 723–741
Preview abstract
Understanding the broader landscape of potential harms from algorithmic systems enables practitioners to better anticipate consequences of the systems they build. It also supports the prospect of incorporating controls to help minimize harms that emerge from the interplay of technologies and social and cultural dynamics. A growing body of scholarship has identified a wide range of harms across different algorithmic and machine learning (ML) technologies. However, computing research and practitioners lack a high level and synthesized overview of harms from algorithmic systems arising at the micro-, meso-, and macro-levels of society. We present an applied taxonomy of sociotechnical harms to support more systematic surfacing of potential harms in algorithmic systems. Based on a scoping review of prior research on harms from AI systems (n=172), we identified five major themes related to sociotechnical harms — allocative, quality-of-service, representational, social system, and interpersonal harms. We describe these categories of harm, and present case studies that illustrate the usefulness of the taxonomy. We conclude with a discussion of challenges and under-explored areas of harm in the literature, which present opportunities for future research.
View details
Preview abstract
How reliably can we trust the scores obtained from social bias benchmarks as faithful indicators of problematic social biases in a given model?
In this work we study this question by contrasting social biases with \underline{non}-social biases that might not even be discernible to human eye. To do so, empirically we simulate various alternative constructions for a given benchmark based on innocuous modifications. (such as paraphrasing or random-sampling) that maintain the essence of their social bias.
On two well-known social bias benchmarks (Winogender(Rudinger et al, 2019) and BiasNLI(Dev et al 2020)) we observe that the choice of these shallow modifications have surprising effect in the resulting degree of bias across various models.
We hope these troubling observations motivates more robust measures of social biases.
View details