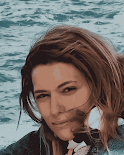
Syrine Krichene
Syrine Krichene is at Google Research Zurich, where she works on developing efficient systems for NLP and Sequential Question Answering. Prior to joining Google, Syrine worked as a machine learning researcher at Criteo, on optimization and recommender systems, and as a visiting scholar at UC Berkeley where she worked on optimization and game theory.
Syrine obtained her BS and MS degrees in Applied Mathematics and Computer Science from INP-ENSIMAG France.
Research Areas
Authored Publications
Google Publications
Other Publications
Sort By
MatCha: Enhancing Visual Language Pretraining with Math Reasoning and Chart Derendering
Chenxi Pang
Mandar Joshi
Nigel Collier
Under review (2022)
Preview abstract
Visual language data such as plots, charts, and infographics are ubiquitous in the human world. However, state-of-the-art vision-language models do not perform well on these data. We propose a set of pretraining tasks to enhance visual language models' capabilities in jointly modeling charts/plots and language data. We initialize with Pix2Struct, a recently proposed image-to-text visual language model and continue pretraining with our proposed objectives. We argue that numerical reasoning and plot deconstruction enable a model with the key capabilities of (1) extracting key information and (2) reasoning on the extracted information. On standard benchmarks such as PlotQA and ChartQA, our continually pretrained MatCha model outperforms state-of-the-art methods by as much as ~20%. We also examine how well does MatCha pretraining transfer to domains such as screenshot, textbook, and poster figures. We observe improvement over the base Pix2Struct checkpoint by 1.2% on average, verifying the usefulness of MatCha pretraining on broader visual language tasks.
View details
DePlot: One-shot visual language understanding by plot-to-text translation
Chenxi Pang
Mandar Joshi
Nigel Collier
Under review (2022)
Preview abstract
Visual language such as charts and plots are ubiquitous in the human world. Comprehending plots and charts requires strong reasoning skills. Prior state-of-the-art models are end-to-end multimodal Transformers pretrained with dedicated plot derendering and numerical reasoning objectives. However, the models reasoning capabilities still fall short and will generally fail on complex queries.
In this paper, we decompose the multimodal reasoning problem into first, a modality conversion problem from image to text, then a purely textual reasoning problem. Through combining a pretrained image-to-text model and an LLM for the task of chart/figure reasoning. Compared with a SOTA model finetuned on >10k data points, our plug-and-play model DePlot-LLM achieves >20% improvement over finetuned SOTA with just one-shot prompting.
View details
Table-To-Text generation and pre-training with TabT5
Ewa Andrejczuk
Findings of EMNLP (2022)
Preview abstract
Encoder-only transformer models have been successfully applied to different table understanding tasks, as in TAPAS (Herzig et al., 2020). A major limitation of these architectures is that they are constrained to classification-like tasks such as cell selection or entailment detection. We present TABT5, an encoder-decoder model that generates natural language text based on tables and textual inputs. TABT5, overcomes the encoder-only limitation by incorporating a decoder component and leverages the input structure with table specific embeddings as well as pre-training. TABT5 achieves new state-of-the-art results on several domains, including spreadsheet formula prediction (15% increase in sequence accuracy), question answering (10% increase in sequence accuracy) and data-to-text generation (2% increas in BLEU).
View details
TAPAS at SemEval-2021 Task 9: Reasoning over tables with intermediate pre-training
Thomas Müller
Proceedings of the Fifteenth Workshop on Semantic Evaluation (2021)
Preview abstract
We present the TAPAS contribution to the Shared Task on Statement Verification and Evidence Finding with Tables (SemEval 2021 Task 9, Wang et al. (2021)). SEM TAB FACT Task A is a classification task of recognizing if a statement is entailed, neutral or refuted by the content of a given table. We adopt the binary TAPAS model of Eisenschlos et al. (2020) to this task. We learn two binary classification models: A first model to predict if a statement is neutral or non-neutral and a second one to predict if it is entailed or refuted. As the shared task training set contains only entailed or refuted examples, we generate artificial neutral examples to train the first model. Both models are pre-trained using a MASKLM objective, intermediate counter-factual and synthetic data (Eisenschlos et al., 2020) and TABFACT (Chen et al., 2020), a large table entailment dataset. We find that the artificial neutral examples are somewhat effective at training the first model, achieving 68.03 test F1 versus the 60.47 of a majority baseline. For the second stage, we find that the pre-training on the intermediate data and TABFACT improves the results over MASKLM pre-training (68.03 vs 57.01).
View details
Preview abstract
Recent advances in open-domain QA have led to strong models based on dense retrieval, but only focused on retrieving textual passages. In this work, we tackle open-domain QA over tables for the first time, and show that retrieval can be improved by a retriever designed to handle tabular context. We present an effective pre-training procedure for our retriever and improve retrieval quality with mined hard negatives. As relevant datasets are missing, we extract a subset of NATURAL QUESTIONS (Kwiatkowski et al., 2019) into a Table QA dataset. We find that our retriever improves retrieval results from 72.0 to 81.1 recall@10 and end-to-end QA results from 33.8 to 37.7 exact match, over a BERT based retriever
View details
DoT: An efficient Double Transformer for NLP tasks with tables
Thomas Müller
Findings of the Association for Computational Linguistics: ACL 2021
Preview abstract
Transformer-based approaches have been successfully used to obtain state-of-the-art accuracy on natural language processing (NLP) tasks with semi-structured tables. These model architectures are typically deep, resulting in slow training and inference, especially for long inputs. To improve efficiency while maintaining a high accuracy, we propose a new architecture, DoT, a double transformer model, that decomposes the problem into two sub-tasks: A shallow pruning transformer that selects the top-K tokens, followed by a deep task-specific transformer that takes as input those K tokens. Additionally, we modify the task-specific attention to incorporate the pruning scores. The two transformers are jointly trained by optimizing the task-specific loss. We run experiments on three benchmarks, including entailment and question-answering. We show that for a small drop of accuracy, DoT improves training and inference time by at least 50%. We also show that the pruning transformer effectively selects relevant tokens enabling the end-to-end model to maintain similar accuracy as slower baseline models. Finally, we analyse the pruning and give some insight into its impact on the task model.
View details
Preview abstract
Table entailment, the binary classification task of finding if a sentence is supported or refuted by the content of a table, requires understanding language and table structure as well as numerical and discrete reasoning. While there is extensive work on textual entailment, table entailment is less well studied. We adapt TAPAS (Herzig et al., 2020), a table-based BERT model, to recognize entailment.
Motivated by the benefits of data augmentation, we create a balanced dataset of millions of automatically created training examples which are learned in an intermediate step prior to fine-tuning. This new data is not only useful for table entailment, but also for SQA (Iyyer et al., 2017), a sequential table QA task. To be able to use long examples as input of BERT models, we evaluate table pruning techniques as a pre-processing step to drastically improve the training and prediction efficiency at a moderate drop in accuracy. The different methods set the new state0o-of-the-art on the TabFact (Chen et al., 2020) and SQA datasets.
View details
No Results Found