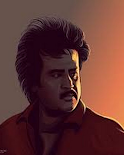
Murali Karthick Baskar
Improving low resource ASR.
Research Areas
Authored Publications
Google Publications
Other Publications
Sort By
Preview abstract
Hard and soft distillation are two popular approaches for knowledge distillation from a teacher to student ASR model. Despite soft distillation being better than hard distillation, it has several limitations. First, training convergence depends on the match between the teacher and student alignments. Second, soft distillation suffers quality regressions when using teacher and student models with different architectures. Third, in case of non-causal teacher models, soft distillation requires tuning of the shift in teacher alignments to the right. Finally, soft distillation requires both the teacher and student models to have the same temporal sampling rates. In this work, we propose a novel knowledge distillation method for RNN-T models that tackles limitations of both hard and soft distillation approaches. We call our method Full-sum distillation, which simply distills the sequence posterior probability of the teacher model to the student model. Thus, this method does not depend directly on the noisy labels to distill knowledge as well as it does not depend on time dimension. We also propose a variant of Full-sum distillation to distill the sequence discriminative knowledge of the teacher model to the student model to further improve performance. Using full-sum distillation, we achieve significant improvements when training with strong and weak teacher models on public data as well as on in-house production data.
View details
Reducing domain mismatch in self-supervised speech pretraining
Yu Zhang
submission to Interspeech 2022 (2022) (to appear)
Preview abstract
Masked speech modeling (MSM) methods such as wav2vec2 or w2v-BERT learn representations over speech frames which are randomly masked within an utterance. While these methods improve performance of Automatic Speech Recognition (ASR) systems, they have one major limitation. They treat all unsupervised speech samples with equal weight, which hinders learning as not all samples have relevant information to learn meaningful representations. In this work, we address this limitation. We propose ask2mask (ATM), a novel approach to focus on specific samples during MSM pre-training. ATM employs an external ASR model or scorer to weight unsupervised input samples in two different ways: 1) A fine-grained data selection is performed by masking over the highly confident input frames as
chosen by the scorer. This allows the model to learn meaningful representations. 2) ATM is further extended to focus at utterancelevel by weighting the final MSM loss with the utterancelevel confidence score. We conduct fine-tuning experiments on two well-benchmarked corpora: LibriSpeech (matching the pretraining data) and Commonvoice, TED-LIUM, AMI and CHiME6 (not matching the pre-training data). The results substantiate the efficacy of ATM on significantly improving the recognition
performance under mismatched conditions (up to 11.6% relative over published results and upto 4.46% relative over our internal baseline) while still yielding modest improvements under matched
conditions.
View details
Ask2Mask: Guided Data Selection for Masked Speech Modeling
Pedro Jose Moreno Mengibar
Yu Zhang
IEEE Journal of Selected Topics in Signal Processing (2022)
Preview abstract
Masked speech modeling (MSM) pre-training methods such as wav2vec2 or w2v-BERT randomly mask speech frames in an utterance and compute losses on the masked instances. While these methods improve performance of Automated Speech Recognition (ASR) systems, they have one major limitation. They generally perform best under matched conditions, i.e., when the data used for pre-training is matched to the data used for fine-tuning. Using out-of-domain (OOD) pre-training data with limited in-domain fine-tuning data from the target domain results in reduced gains. The relative value of in-domain data within a MSM pre-training corpus has not been well-explored in the literature. In this work, we address precisely this limitation. We propose ask2mask, a novel approach to focus on samples relevant to the target domain (in-domain) during pre-training with OOD or any available data. To perform this fine-grained data selection, ATM applies masking only to input frames with high confidence scores obtained from an external classification model. This allows the model to achieve meaningful in-domain representations and simultaneously discard low-confidence frames which could lead to learning erroneous representations. The ATM approach is further extended to focus on utterances with high confidences by scaling the final MSM loss computed for each masked input frame with the utterance-level confidence score. We conduct experiments on two well-benchmarked read speech corpus (Librispeech) and conversational speech corpus (AMI). The results substantiate the efficacy of ATM on significantly improving target domain performance under mismatched conditions while still yielding modest improvements under matched conditions.
View details
No Results Found