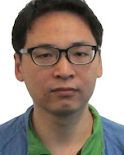
Junfeng He
Short bio
Junfeng He is a research scientist in Google Research. He got his bachelor and master degree from Tsinghua University, and PhD from Columbia University.His full publication list can be found in google scholar page
Research areas
His major research areas include computer vision, machine learning, search/retrieval/ranking, HCI, and health. He has >15 years research experience on image retrieval&classification, image editing and its detection, ranking, large scale (approximate) machine learning, etc.His current research interest is the intersection of computer vision and human vision/perception, for instance
Recent research papers
Modeling of gaze tracking and its applications
Modeling of human attention/perception/vision and its applications
Leverage human vision/perception to improve computer vision
Related trustworthy ML problems
Media coverage
Leverage human attention/saliency models to improve JPEG XL compression
Awards
Authored Publications
Google Publications
Other Publications
Sort By
Accelerating eye movement research via accurate and affordable smartphone eye tracking
Na Dai
Ethan Steinberg
Kantwon Rogers
Venky Ramachandran
Mina Shojaeizadeh
Li Guo
Nature Communications, vol. 11 (2020)
Preview abstract
Eye tracking has been widely used for decades in vision research, language and usability. However, most prior research has focused on large desktop displays using specialized eye trackers that are expensive and cannot scale. Little is known about eye movement behavior on phones, despite their pervasiveness and large amount of time spent. We leverage machine learning to demonstrate accurate smartphone-based eye tracking without any additional hardware. We show that the accuracy of our method is comparable to state-of-the-art mobile eye trackers that are 100x more expensive. Using data from over 100 opted-in users, we replicate key findings from previous eye movement research on oculomotor tasks and saliency analyses during natural image viewing. In addition, we demonstrate the utility of smartphone-based gaze for detecting reading comprehension difficulty. Our results show the potential for scaling eye movement research by orders-of-magnitude to thousands of participants (with explicit consent), enabling advances in vision research, accessibility and healthcare.
View details
On-device Few-shot Personalization for Real-time Gaze Estimation
Khoi Pham
Chase Riley Roberts
Dmitry Lagun
ICCV 2019 Gaze workshop
Preview abstract
Recent research has demonstrated the ability to estimate user’s gaze on mobile devices, by performing inference from an image captured with the phone’s front-facing camera, and without requiring specialized hardware. Gaze estimation accuracy is known to improve with additional calibration data from the user. However, most existing methods require either significant number of calibration
points or computationally intensive model fine-tuning that is practically infeasible on a mobile device. In this paper, we overcome limitations of prior work by proposing a novel few-shot personalization approach for 2D gaze estimation. Compared to the best calibration-free model [11], the proposed method yields substantial improvements in gaze prediction accuracy (24%) using only 3 calibration
points in contrast to previous personalized models that offer less improvement while requiring more calibration points. The proposed model requires 20x fewer FLOPS than the state-of-the-art personalized model [11] and can be run entirely on-device and in real-time, thereby unlocking a variety of important applications like accessibility, gaming and human-computer interaction.
View details
No Results Found