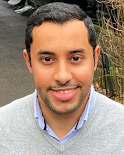
Antonious M. Girgis
Antonious M. Girgis is currently a research scientist at Google, Mountain View, CA, USA. He received the B.Sc. degree in electrical engineering from Cairo University, Egypt, in 2014, the M.Sc. degree in electrical engineering from Nile University, Egypt, in 2018, and the Ph.D. degree in the electrical and computer engineering from the University of California, Los Angeles (UCLA), in 2023. He was the receipt of the 2021 ACM Conference on Computer and Communications Security (CCS) best paper award, and the receipt of distinguished Ph.D. dissertation award in signals and systems from the ECE department, UCLA. He was an Exchange Research Assistant with Sabanci University, Turkey, from 2016 to 2017. He received the Masters Fellowship and a graduate Research Assistantship from Nile University for the years 2014-2018. He received the Electrical and Computer Engineering Department Fellowship from UCLA for the year 2018/2019, and the 2022 Amazon Ph.D fellowship. His research interests include privacy, machine learning, information theory, and optimization.
Research Areas
Authored Publications
Google Publications
Other Publications
Sort By
Learning from straggler clients in federated learning
Ehsan Amid
Rohan Anil
Arxiv (2024) (to appear)
Preview abstract
How well do existing federated learning algorithms learn from client devices that return model updates with a significant time delay? Is it even possible to learn effectively from clients that report back minutes, hours, or days after being scheduled? We answer these questions by developing Monte Carlo simulations of client latency that are guided by real-world applications. We compare well-known synchronous optimization algorithms like FedAvg and FedAdam with the state-of-the-art asynchronous FedBuff algorithm, and discover that these existing approaches often struggle to learn from severely delayed clients. To improve upon these, we experiment with modifications including distillation regularization and exponential moving averages of model weights. Finally, we invent two new algorithms, FARe-DUST and FeAST-on-MSG, based on distillation and averaging, respectively. Experiments with the EMNIST, CIFAR-100, and StackOverflow benchmark federated learning tasks demonstrate that our new algorithms outperform existing ones in terms of accuracy for straggler clients, while also providing better trade-offs between training time and total accuracy.
View details
No Results Found