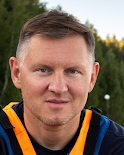
ALEXEY KOLESNIKOV
Research Areas
Authored Publications
Google Publications
Other Publications
Sort By
Improving variant calling using population data and deep learning
Andrew Carroll
Nae-Chyun Chen
Sidharth Goel
BMC Bioinformatics (2023)
Preview abstract
Large-scale population variant data is often used to filter and aid interpretation of variant calls in a single sample. These approaches do not incorporate population information directly into the process of variant calling, and are often limited to filtering which trades recall for precision. In this study, we develop population-aware DeepVariant models with a new channel encoding allele frequencies from the 1000 Genomes Project. This model reduces variant calling errors, improving both precision and recall in single samples, and reduces rare homozygous and pathogenic clinvar calls cohort-wide. We assess the use of population-specific or diverse reference panels, finding the greatest accuracy with diverse panels, suggesting that large, diverse panels are preferable to individual populations, even when the population matches sample ancestry. Finally, we show that this benefit generalizes to samples with different ancestry from the training data even when the ancestry is also excluded from the reference panel.
View details
Accurate human genome analysis with Element Avidity sequencing
Andrew Carroll
Bryan Lajoie
Daniel Cook
Kelly N. Blease
Kishwar Shafin
Lucas Brambrink
Maria Nattestad
Semyon Kruglyak
bioRxiv (2023)
Preview abstract
We investigate the new sequencing technology Avidity from Element Biosciences. We show that Avidity whole genome sequencing matches mapping and variant calling accuracy with Illumina at high coverages (30x-50x) and is noticeably more accurate at lower coverages (20x-30x). We quantify base error rates of Element reads, finding lower error rates, especially in homopolymer and tandem repeat regions. We use Element’s ability to generate paired end sequencing with longer insert sizes than typical short–read sequencing. We show that longer insert sizes result in even higher accuracy, with long insert Element sequencing giving noticeably more accurate genome analyses at all coverages.
View details
Approximate haplotagging with DeepVariant
Daniel Cook
Maria Nattestad
John E. Gorzynski
Sneha D. Goenka
Euan Ashley
Miten Jain
Karen Miga
Benedict Paten
Andrew Carroll
Kishwar Shafin
bioRxiv (2023)
Preview abstract
Long-read sequencing technology has enabled variant detection in difficult-to-map regions of the genome and enabled rapid genetic diagnosis in clinical settings. Rapidly evolving third-
generation sequencing like Pacific Biosciences (PacBio) and Oxford nanopore technologies (ONT) are introducing newer platforms and data types. It has been demonstrated that variant calling methods based on deep neural networks can use local haplotyping information with long-reads to improve the genotyping accuracy. However, using local haplotype information creates an overhead as variant calling needs to be performed multiple times which ultimately makes it difficult to extend to new data types and platforms as they get introduced. In this work, we have developed a local haplotype approximate method that enables state-of-the-art variant calling performance with multiple sequencing platforms including PacBio revio platfrom, ONT R10.4 simplex and duplex data. This addition of local haplotype approximation makes DeepVariant a universal variant calling solution for all long-read sequencing platforms.
View details
A draft human pangenome reference
Wen-Wei Liao
Mobin Asri
Jana Ebler
Daniel Doerr
Marina Haukness
Shuangjia Lu
Julian K. Lucas
Jean Monlong
Haley J. Abel
Silvia Buonaiuto
Xian Chang
Haoyu Cheng
Justin Chu
Vincenza Colonna
Jordan M. Eizenga
Xiaowen Feng
Christian Fischer
Robert S. Fulton
Shilpa Garg
Cristian Groza
Andrea Guarracino
William T. Harvey
Simon Heumos
Kerstin Howe
Miten Jain
Tsung-Yu Lu
Charles Markello
Fergal J. Martin
Matthew W. Mitchell
Katherine M. Munson
Moses Njagi Mwaniki
Adam M. Novak
Hugh E. Olsen
Trevor Pesout
David Porubsky
Pjotr Prins
Jonas A. Sibbesen
Jouni Sirén
Chad Tomlinson
Flavia Villani
Mitchell R. Vollger
Lucinda L Antonacci-Fulton
Gunjan Baid
Carl A. Baker
Anastasiya Belyaeva
Konstantinos Billis
Andrew Carroll
Sarah Cody
Daniel Cook
Robert M. Cook-Deegan
Omar E. Cornejo
Mark Diekhans
Peter Ebert
Susan Fairley
Olivier Fedrigo
Adam L. Felsenfeld
Giulio Formenti
Adam Frankish
Yan Gao
Nanibaa’ A. Garrison
Carlos Garcia Giron
Richard E. Green
Leanne Haggerty
Kendra Hoekzema
Thibaut Hourlier
Hanlee P. Ji
Eimear E. Kenny
Barbara A. Koenig
Jan O. Korbel
Jennifer Kordosky
Sergey Koren
HoJoon Lee
Alexandra P. Lewis
Hugo Magalhães
Santiago Marco-Sola
Pierre Marijon
Ann McCartney
Jennifer McDaniel
Jacquelyn Mountcastle
Maria Nattestad
Sergey Nurk
Nathan D. Olson
Alice B. Popejoy
Daniela Puiu
Mikko Rautiainen
Allison A. Regier
Arang Rhie
Samuel Sacco
Ashley D. Sanders
Valerie A. Schneider
Baergen I. Schultz
Kishwar Shafin
Michael W. Smith
Heidi J. Sofia
Ahmad N. Abou Tayoun
Francoise Thibauld-Nissen
Francesa Floriana Tricomi
Justin Wagner
Brian Walenz
Jonathan M. D. Wood
Aleksey V. Zimin
Guillaume Borque
Mark J. P. Chaisson
Paul Flicek
Adam M. Phillippy
Justin Zook
Evan E. Eichler
David Haussler
Ting Wang
Erich D. Jarvis
Karen H. Miga
Glenn Hickey
Erik Garrison
Tobias Marschall
Ira M. Hall
Heng Li
Benedict Paten
Nature (2023)
Preview abstract
Here the Human Pangenome Reference Consortium presents a first draft of the human pangenome reference. The pangenome contains 47 phased, diploid assemblies from a cohort of genetically diverse individuals. These assemblies cover more than 99% of the expected sequence in each genome and are more than 99% accurate at the structural and base pair levels. Based on alignments of the assemblies, we generate a draft pangenome that captures known variants and haplotypes and reveals new alleles at structurally complex loci. We also add 119 million base pairs of euchromatic polymorphic sequences and 1,115 gene duplications relative to the existing reference GRCh38. Roughly 90 million of the additional base pairs are derived from structural variation. Using our draft pangenome to analyse short-read data reduced small variant discovery errors by 34% and increased the number of structural variants detected per haplotype by 104% compared with GRCh38-based workflows, which enabled the typing of the vast majority of structural variant alleles per sample.
View details
Improving variant calling using population data and deep learning
Nae-Chyun Chen
Sidharth Goel
Andrew Carroll
BMC Bioinformatics (2023)
Preview abstract
Large-scale population variant data is often used to filter and aid interpretation of variant calls in a single sample. These approaches do not incorporate population information directly into the process of variant calling, and are often limited to filtering which trades recall for precision. In this study, we develop population-aware DeepVariant models with a new channel encoding allele frequencies from the 1000 Genomes Project. This model reduces variant calling errors, improving both precision and recall in single samples, and reduces rare homozygous and pathogenic clinvar calls cohort-wide. We assess the use of population-specific or diverse reference panels, finding the greatest accuracy with diverse panels, suggesting that large, diverse panels are preferable to individual populations, even when the population matches sample ancestry. Finally, we show that this benefit generalizes to samples with different ancestry from the training data even when the ancestry is also excluded from the reference panel.
View details
How DeepConsensus Works
Aaron Wenger
Anastasiya Belyaeva
Andrew Carroll
Armin Töpfer
Ashish Teku Vaswani
Daniel Cook
Felipe Llinares
Gunjan Baid
Howard Yang
Jean-Philippe Vert
Kishwar Shafin
Maria Nattestad
Waleed Ammar
William J. Rowell
(2022)
Preview abstract
N/A
These are slides for a public video about DeepConsensus
View details
DeepConsensus improves the accuracy of sequences with a gap-aware sequence transformer
Aaron Wenger
Andrew Walker Carroll
Armin Töpfer
Ashish Teku Vaswani
Daniel Cook
Felipe Llinares
Gunjan Baid
Howard Cheng-Hao Yang
Jean-Philippe Vert
Kishwar Shafin
Maria Nattestad
Waleed Ammar
William J. Rowell
Nature Biotechnology (2022)
Preview abstract
Genomic analysis requires accurate sequencing in sufficient coverage and over difficult genome regions. Through repeated sampling of a circular template, Pacific Biosciences developed long (10-25kb) reads with high overall accuracy, but lower homopolymer accuracy. Here, we introduce DeepConsensus, a transformer-based approach which leverages a unique alignment loss to correct sequencing errors. DeepConsensus reduces errors in PacBio HiFi reads by 42%, compared to the current approach. We show this increases the yield of PacBio HiFi reads at Q20 by 9%, at Q30 by 27%, and at Q40 by 90%. With two SMRT cells of HG003, reads from DeepConsensus improve hifiasm assembly contiguity (NG50 4.9Mb to 17.2Mb), increase gene completeness (94% to 97%), reduce false gene duplication rate (1.1% to 0.5%), and improve assembly base accuracy (QV43 to QV45), and also reduce variant calling errors by 24%.
View details
precisionFDA Truth Challenge V2: Calling variants from short- and long-reads in difficult-to-map regions.
Andrew Carroll
Gunjan Baid
Howard Yang
Maria Nattestad
Sidharth Goel
Cell Genomics (2022)
Preview abstract
The precisionFDA Truth Challenge V2 aimed to assess the state-of-the-art of variant calling in difficult-to-map regions and the Major Histocompatibility Complex (MHC). Starting with fastq files, 20 challenge participants applied their variant calling pipeline and submitted 64 variant callsets for one or more sequencing technologies (~35X Illumina, ~35X PacBio HiFi, and ~50X Oxford Nanopore Technologies). Submissions were evaluated following best practices for benchmarking small variants with the new GIAB benchmark sets and genome stratifications. Challenge submissions included a number of innovative methods for all three technologies, with graph-based methods and machine-learning methods scoring best for short-reads and long-read datasets, respectively. New methods out-performed the winners of the 2016 Truth Challenge across technologies, and new machine-learning approaches combining multiple sequencing technologies performed particularly well. Recent developments in sequencing and variant calling have enabled benchmarking variants in challenging genomic regions, paving the way for the identification of previously unknown clinically relevant variants.
View details
Technical development of rapid whole genome nanopore sequencing and variant identification pipeline
Andrew Carroll
Ankit Sethia
Benedict Paten
Christopher Wright
Daniel R Garalde
Dianna G. Fisk
Elizabeth Spiteri
Euan Ashley
Fritz J. Sedlazeck
Gunjan Baid
Jean Monlong
Jeffrey W Christle
John E. Gorzynski
Jonathan A Bernstein
Joseph Guillory
Karen P. Dalton
Katherine Xiong
Kishwar Shafin
Maria Nattestad
Maura RZ Ruzhnikov
Megan E. Grove
Mehrzad Samadi
Miten Jain
Sneha D. Goenka
Tanner D. Jensen
Tong Zhu
Trevor Pesout
Nature Biotechnology (2022)
Preview abstract
Whole genome sequencing can identify pathogenic variants for genetic disease but the time
required for sequencing and analysis has been a barrier to its use in acutely ill patients. Here,
we develop an approach to ultra-rapid nanopore whole genome sequencing that combines an
efficient sample preparation protocol, distributed sequencing over 48 flow cells, near real-time
base calling and alignment, accelerated variant calling, and fast variant filtration. We show
that this framework provides accurate variant prioritization in less than half the fastest time
recorded for an equivalent analysis to date.
View details
Ultra-rapid whole genome nanopore sequencing in a critical care setting
Andrew Carroll
Ankit Sethia
Benedict Paten
Christopher Wright
Courtney J. Wusthoff
Daniel R Garalde
Dianna G. Fisk
Elizabeth Spiteri
Euan Ashley
Fritz J. Sedlazeck
Gunjan Baid
Henry Chubb
Jeffrey W Christle
Jeffrey W. Christle
John E. Gorzynski
Jonathan A Bernstein
Joseph Guillory
Joshua W. Knowles
Katherine Xiong
Kishwar Shafin
Kyla Dunn
Marco Perez
Maria Nattestad
Maura RZ Ruzhnikov
Megan E. Grove
Mehrzad Samadi
Michael Ma
Miten Jain
Scott R. Ceresnak
Sneha D. Goenka
Tanner D. Jensen
Tia Moscarello
Tong Zhu
Trevor Pesout
New England Journal of Medicine (2022)
Preview abstract
Background
Genetic disease is a major contributor to critical care hospitalization, especially in younger patients. While early genetic diagnosis can guide clinical management, the turnaround time for whole genome based diagnostic testing has traditionally been measured in months. Recent programs in neonatal populations have reduced turnaround time into the range of days and shown that rapid genetic diagnosis enhances patient care and reduces healthcare costs. Yet, most decisions in critical care need to be made on hourly timescales.
Methods
We developed a whole genome sequencing approach designed to provide a genetic diagnosis within hours. Optimized highly parallel nanopore sequencing was coupled to a high-performance cloud compute system to implement near real-time basecalling and alignment followed by accelerated central and graphics processor unit variant calling. A custom scheme for variant prioritization took only minutes to rank variants most likely to be deleterious allowing efficient manual review and classification according to American College of Medical Genetics and Genomics guidelines.
Results
We performed whole genome sequencing on 12 patients from the critical care units of Stanford hospitals. In 10 cases, the pipeline produced diagnostic results faster than all previously published clinical genome analyses. Per patient, DNA extraction, library preparation, and nanopore sequencing across 48 flow cells generated 173–236 GigaBases of sequencing data in as little as 1:50 hours. After optimization, the average turnaround time was 7:58 hours (range 7:18–9:0 hours). A pathogenic or likely pathogenic variant was identified in five out of 12 patients (42%). After Sanger or short read sequencing confirmation in a CLIA-approved laboratory, this validated diagnosis altered clinical management in every case.
Conclusions
We developed an approach to make a genetic diagnosis from whole genome sequencing in hours, returning actionable, cost-saving diagnostic information on critical care timescales.
View details
Knowledge distillation for fast and accurate DNA sequence correction
Anastasiya Belyaeva
Daniel Cook
Kishwar Shafin
Daniel Liu
Armin Töpfer
Aaron Wenger
William J. Rowell
Howard Yang
Andrew Carroll
Maria Nattestad
Learning Meaningful Representations of Life (LMRL) Workshop NeurIPS 2022
Preview abstract
Accurate genome sequencing can improve our understanding of biology and the genetic basis of disease. The standard approach for generating DNA sequences from PacBio instruments relies on HMM-based models. Here, we introduce Distilled DeepConsensus - a distilled transformer–encoder model for sequence correction, which improves upon the HMM-based methods with runtime constraints in mind. Distilled DeepConsensus is 1.3x faster and 1.5x smaller than its larger counterpart while improving the yield of high quality reads (Q30) over the HMM-based method by 1.69x (vs. 1.73x for larger model). With improved accuracy of genomic sequences, Distilled DeepConsensus improves downstream applications of genomic sequence analysis such as reducing variant calling errors by 39% (34% for larger model) and improving genome assembly quality by 3.8% (4.2% for larger model). We show that the representations learned by Distilled DeepConsensus are similar between faster and slower models.
View details
Haplotype-aware variant calling enables high accuracy in nanopore long-reads using deep neural networks
Kishwar Shafin
Trevor Pesout
Maria Nattestad
Sidharth Goel
Gunjan Baid
Mikhail Kolmogorov
Jordan M. Eizenga
Karen Miga
Paolo Carnevali
Miten Jain
Andrew Carroll
Benedict Paten
Nature Methods (2021)
Preview abstract
Long-read sequencing has the potential to transform variant detection by reaching currently difficult-to-map regions and routinely linking together adjacent variations to enable read-based phasing. Third-generation nanopore sequence data have demonstrated a long read length, but current interpretation methods for their novel pore-based signal have unique error profiles, making accurate analysis challenging. Here, we introduce a haplotype-aware variant calling pipeline, PEPPER-Margin-DeepVariant, that produces state-of-the-art variant calling results with nanopore data. We show that our nanopore-based method outperforms the short-read-based single-nucleotide-variant identification method at the whole-genome scale and produces high-quality single-nucleotide variants in segmental duplications and low-mappability regions where short-read-based genotyping fails. We show that our pipeline can provide highly contiguous phase blocks across the genome with nanopore reads, contiguously spanning between 85% and 92% of annotated genes across six samples. We also extend PEPPER-Margin-DeepVariant to PacBio HiFi data, providing an efficient solution with superior performance over the current WhatsHap-DeepVariant standard. Finally, we demonstrate de novo assembly polishing methods that use nanopore and PacBio HiFi reads to produce diploid assemblies with high accuracy (Q35+ nanopore-polished and Q40+ PacBio HiFi-polished).
View details
Preview abstract
In this blog, we discuss a new channel in DeepVariant which encodes haplotype information in long-read data, and was released with DeepVariant v1.1. We review how haplotypes relate to variant calling, show examples improved by the channel, and quantify the accuracy improvement with PacBio HiFi.
View details
DeepTrio: Variant Calling in Families Using Deep Learning
Gunjan Baid
Howard Yang
Maria Nattestad
Sidharth Goel
bioRxiv (2021)
Preview abstract
Every human inherits one copy of the genome from their mother and another from their father. Parental inheritance helps us understand the transmission of traits and genetic diseases, which often involve de novo variants and rare recessive alleles. Here we present DeepTrio, which learns to analyze child-mother-father trio from the joint sequence information, without explicit encoding of inheritance priors. DeepTrio to learn how to weigh sequencing error, mapping error, and de novo rates and genome context directly from the sequence data. DeepTrio has higher accuracy on both Illumina and PacBio HiFi data when compared to DeepVariant. Improvements are especially pronounced at lower coverages (with 20x DeepTrio roughly equivalent to 30x DeepVariant). As DeepTrio learns directly from data, we also demonstrate extensions to exome calling and calling with duos (child and one parent) solely by changing the training data. DeepTrio includes pre-trained models for Illumina WGS, Illumina exome, and PacBio HiFi.
View details
A population-specific reference panel for improved genotype imputation in African Americans
Jared O’Connell
Meghan Moreno
Helen Li
Nadia Litterman
Elizabeth Noblin
Anjali Shastri
Elizabeth H. Dorfman
Suyash Shringarpure
23andMe Research Team
Adam Auton
Andrew Carroll
Communications Biology (2021)
Preview abstract
There is currently a dearth of accessible whole genome sequencing (WGS) data for individuals residing in the Americas with Sub-Saharan African ancestry. We generated whole genome sequencing data at intermediate (15×) coverage for 2,294 individuals with large amounts of Sub-Saharan African ancestry, predominantly Atlantic African admixed with varying amounts of European and American ancestry. We performed extensive comparisons of variant callers, phasing algorithms, and variant filtration on these data to construct a high quality imputation panel containing data from 2,269 unrelated individuals. With the exception of the TOPMed imputation server (which notably cannot be downloaded), our panel substantially outperformed other available panels when imputing African American individuals. The raw sequencing data, variant calls and imputation panel for this cohort are all freely available via dbGaP and should prove an invaluable resource for further study of admixed African genetics.
View details
DeepVariant over the years
Andrew Carroll
Daniel Cook
Gunjan Baid
Howard Yang
Maria Nattestad
(2021)
Preview abstract
The development of DeepVariant was motivated by the following question: if computational biologists can look at pileup images of reads to identify variants, can we train an image classification model to perform this task? To answer this question, we began working on DeepVariant in 2015, and the first open-source version (v0.4) of the software was released in late 2017. Since v0.4, the project has come a long way, and there have been eight additional releases. We originally began development on Illumina whole-genome sequencing (WSG) data, and the first release included one model for this data type. Over the years, we have added support for additional sequencing technologies, and we now provide models for Illumina whole-exome sequencing (WES) data, Pacific Bioscience (PacBio) Hifi data, and a hybrid model for Illumina and PacBio WGS data combined. We have also collaborated with a team at UC Santa Cruz to train DeepVariant using Oxford Nanopore data. The resulting tool, PEPPER-DeepVariant, uses PEPPER to generate candidates more effectively for Nanopore data. In addition to new models, new capabilities have been added, such as the best practices for cohort calling in v0.9 and DeepTrio, a trio and duo caller, in v1.1. For each release, we focus on building highly-accurate models, reducing runtime, and improving the user experience. In this post, we summarize the improvements in accuracy and runtime over the years and highlight a few categories of changes that have led to these improvements.
View details
Preview abstract
Exome and genome sequencing typically use a reference genome to map reads and call variants against. Many (if not a majority) of clinical and research workflows use the prior version of the human reference genome (GRCh37), although an updated and more complete version (GRCh38) was produced in 2013.
We present a method that identifies potential artifacts when using one reference relative to a different reference. We simulate error-free reads from GRCh37 and GRCh38, and map and call variants from one read set to the opposite reference.
When simulated reads are analyzed relative to their own reference, there are no variants called on GRCh37 and 14 on GRCh38. However, when GRCh38 reads are analyzed on GRCh37, there are 69,720 heterozygous variants called with GATK4-HC. Since the reference is monoploid, a heterozygous call is likely an artifact.
Inspection suggests these represent segmental duplications captured in GRCh38, but excluded or collapsed in GRCh37. Some overlap with common resources: 32,688 are present in dbSNP, 28,830 are present gnomAD (with 25,062 listed as filtered for HWE violation), 19 HET variants and 199 HOM overlap ClinVar. In v3.3.2 Genome in a Bottle, 1,123 of these variants overlap the confident regions for HG002, and they are inconsistently labelled as variants or reference. DeepVariant, which is trained on the truth set, seems to have learned about this variability, allowing some measurement of segmental duplication to be made from its output.
Reverse comparison using GRCh37 reads on GRCh38 finds only 30% as many HET variants. This suggests that migrating workflows to GRCh38 eliminates a number of recurrent artifacts, and could present an additional filtration resource for GRCh37 variant files and annotation resources.
View details
Single Molecule High-Fidelity (HiFi) Sequencing with >10 kb Libraries
Aaron Wenger
Andrew Carroll
Arkarachai Fungtammasan
Chen-Shan Chin
Dario Cantu
David R. Rank
Gregory T. Concepcion
Jue Ruan
Paul Peluso
Richard J. Hall
Sergey Koren
William J. Rowell
Plant and Animal Genomes (2019)
Preview abstract
Recent improvements in sequencing chemistry and instrument performance combine to create a new PacBio data type, Single Molecule High-Fidelity reads (HiFi reads). Increased read length and improvement in library construction enables average read lengths of 10-20 kb with average sequence identity greater than 99% from raw single molecule reads. The resulting reads have the accuracy comparable to short read NGS but with 50-100 times longer read length. Here we benchmark the performance of this data type by sequencing and genotyping the Genome in a Bottle (GIAB) HG0002 human reference sample from the National Institute of Standards and Technology (NIST). We further demonstrate the general utility of HiFi reads by analyzing multiple clones of Cabernet Sauvignon. Three different clones were sequenced, and de novo assembled with the CANU assembly algorithm generating draft assemblies of very high contiguity equal to or better than earlier assembly efforts using PacBio long reads. Using the Cabernet Sauvignon Clone 8 assembly as a reference, we mapped the HiFi reads generated from the Clone 6 and Clone 47 to identify single nucleotide polymorphisms (SNPs) and structural variants (SVs) which are specific to each of the three samples.
View details
Preview abstract
In this blog we discuss the newly published use of Pacbio Circular Consensus Sequencing (CCS) at human genome scale. We demonstrate that DeepVariant trained for this data type achieves similar accuracy to available Illumina genomes, and is the only method to achieve competitive accuracy in Indel calling. Early access to this model is available now by request, and we expect general availability in our next DeepVariant release (v0.8)
View details
Accurate circular consensus long-read sequencing improves variant detection and assembly of a human genome
Aaron M. Wenger
Paul Peluso
William J. Rowell
Richard J. Hall
Gregory T. Concepcion
Jana Ebler
Arkarachai Fungtammasan
Nathan D. Olson
Armin Töpfer
Michael Alonge
Medhat Mahmoud
Yufeng Qian
Chen-Shan Chin
Adam M. Phillippy
Michael C. Schatz
Gene Myers
Mark A. DePristo
Jue Ruan
Tobias Marschall
Fritz J. Sedlazeck
Justin M. Zook
Heng Li
Sergey Koren
Andrew Carroll
David R. Rank
Michael W. Hunkapiller
Nature Biotechnology (2019)
Preview abstract
The DNA sequencing technologies in use today produce either highly accurate short reads or less-accurate long reads. We report the optimization of circular consensus sequencing (CCS) to improve the accuracy of single-molecule real-time (SMRT) sequencing (PacBio) and generate highly accurate (99.8%) long high-fidelity (HiFi) reads with an average length of 13.5 kilobases (kb). We applied our approach to sequence the well-characterized human HG002/NA24385 genome and obtained precision and recall rates of at least 99.91% for single-nucleotide variants (SNVs), 95.98% for insertions and deletions <50 bp (indels) and 95.99% for structural variants. Our CCS method matches or exceeds the ability of short-read sequencing to detect small variants and structural variants. We estimate that 2,434 discordances are correctable mistakes in the ‘genome in a bottle’ (GIAB) benchmark set. Nearly all (99.64%) variants can be phased into haplotypes, further improving variant detection. De novo genome assembly using CCS reads alone produced a contiguous and accurate genome with a contig N50 of >15 megabases (Mb) and concordance of 99.997%, substantially outperforming assembly with less-accurate long reads.
View details
Preview abstract
In this blog we discuss the newly published use of PacBio Circular Consensus Sequencing (CCS) at human genome scale. We demonstrate that DeepVariant trained for this data type achieves similar accuracy to available Illumina genomes, and is the only method to achieve competitive accuracy in Indel calling. Early access to this model is available now by request, and we expect general availability in our next DeepVariant release (v0.8).
View details
Accurate circular consensus long-read sequencing improves variant detection and assembly of a human genome
Aaron Wenger
Andrew Carroll
Arkarachai Fungtammasan
Armin Töpfer
Chen-Shan Chin
David R. Rank
Fritz J. Sedlazeck
Gene Myers
Gregory T. Concepcion
Heng Li
Jana Ebler
Jue Ruan
Justin Zook
Mark DePristo
Medhat Mahmoud
Michael Alonge
Michael C. Schatz
Michael W. Hunkapiller
Nathan D. Olson
Paul Peluso
Richard J. Hall
Sergey Koren
Tobias Marschall
William J. Rowell
Yufeng Qian
Nature Biotechnology (2019)
Preview abstract
The major DNA sequencing technologies in use today produce either highly-accurate short reads or noisy long reads. We develop a protocol based on single-molecule, circular consensus sequencing (CCS) to generate highly-accurate, long reads and apply it to sequence the well-characterized human, HG002/NA24385, to 28-fold coverage with 13.5 kb CCS reads that average 99.5% accuracy. We apply existing tools to comprehensively detect variants, and achieve precision and recall above 99.9% for SNVs, 95.9% for indels, and 95.2% for structural variants. Nearly all (99.6%) variants are phased into haplotypes, which further improves variant detection. De novo assembly produces a highly contiguous and accurate genome with contig N50 above 15 Mb and concordance over Q45 (99.997%). From manual curation of discordances, we estimate 1,283 mistakes in the high-quality Genome in a Bottle benchmark are correctable with CCS reads. With only CCS reads, we match or exceed performance of variant detection with accurate short reads and assembly with noisy long reads.
View details
No Results Found