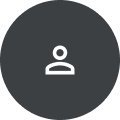
Thibaut Cuvelier
Research Areas
Authored Publications
Google Publications
Other Publications
Sort By
Preview abstract
Middle-mile logistics describes the problem of routing shipments through a network of hubs while respecting deadlines upon arrival. We consider that the hubs are linked by predefined lines, to which we have to assign vehicles. A very challenging aspect of the problem comes from the finite capacity of the vehicles: allocating a shipment to a given vehicle might block another one from using the same vehicle.
Typical exact solution methods, based on a multicommodity-flow formulation, scale poorly with the problem size and real-world instances become quickly intractable. Instead, we turn to reinforcement learning (RL) by rephrasing the middle-mile problem as a multi-objective Markov decision process, where the state is a graph: the lines (edges) between the hubs and the parcels (nodes). At each round, we assign one shipment to a vehicle or decide that it stays in the same hub. The key ingredients of our proposed method are the extraction of small feature graphs from the state and the combination of graph neural networks (GNN) with model-free RL.
We use the PPO (proximal policy optimization) algorithm, which maintains both an actor and a critic, while being able to cope with a varying number of actions depending on the state. We compare linear functions and GraphNet (a particular kind of GNN) to approximate the policy and value functions. GNNs can deliver up to 40% more shipments than a linear function and both approaches scale well with the number of shipments per truck.
View details
Preview abstract
Reinforcement can be a useful tool to solve combinatorial problems, even in the presence of constraints. This presentation details two use cases: one industrial application in the field of logistics, one of a more abstract problem in combinatorial optimization.
View details
OR-Tools' Vehicle Routing Solver: a Generic Constraint-Programming Solver with Heuristic Search for Routing Problems
Steven Agostino Gay
Congrès de la Société française de recherche opérationnelle et d’aide à la décision (ROADEF)(2023) (to appear)
Preview abstract
OR-Tools is the general-purpose optimisation toolbox open-sourced by Google in 2015, being in development since 2008. This toolkit provides a uniform interface to several solvers, both first- and third-party. In particular, it offers a high-level interface for vehicle-routing problems (VRPs). OR-Tools contains several solvers, in particular two CP solvers, CP* (since the first open-source release) and CP-SAT (gold-medal winner at several MiniZinc competitions, developed since 2009), but also two linear solvers: the simplex-based Glop (since 2014), and PDLP, a first-order large-scale linear solver. OR-Tools is being actively developed, with approximately quarterly releases. Outside Google, the solver suite is easily accessible via Google Cloud, either for solving VRPs or mixed-integer linear programs, although the latter API is not yet in general access.
The routing component has historically played a strong role in the development of the overall solver; its major focus is on solving large-scale industrial vehicle-routing problems with complex constraints: vehicle capacities with various starting/ending depots, client time windows considering road traffic and driver breaks, pick-up-and-delivery precedence rules, incompatible shipments within the same vehicle, solution similarity to a previous call to the solver, etc. To this end, a high-level modelling API is proposed to the users in Python, C++, Java, and C#, using only routing concepts, even though the user has access to the underlying constraint-programming model.
From an algorithmic point of view, the routing solver is organised in three parts: (i) first-solution heuristics generate good potential vehicle tours; (ii) local search improves the first solutions, with metaheuristics to guide the search; (iii) a CP engine proves the optimality of the best solution or improves upon it. The main difference with many academic solvers is the focus on generality in the solver, including its heuristics.
View details
À la découverte de Julia !
Datacraft - université Paris-Sorbonne, Sorbonne Center for Artificial Intelligence
4 Pl. Jussieu
75005 Paris
France(2022)
Preview abstract
Dans le bestiaire des langages de programmation, on trouve régulièrement de nouveaux venus, avec un succès souvent très éphémère. La première préversion de Julia est sortie en février 2012, après une incubation dans les laboratoires du MIT. Son objectif est assez simple : fournir une excellente performance à l'exécution du code (en se rapprochant du C), tout en gardant la facilité d'écriture des langages plus dynamiques comme Python ou MATLAB. Plus de dix ans après, le langage et sa communauté se sont développés, la performance est souvent très proche du C (le lecteur de fichiers CSV le plus rapide est d'ailleurs écrit en Julia), les mécanismes de base du langage ont permis de développer une composabilité à grande échelle dans tout l'écosystème (on peut combiner les fonctionnalités de plusieurs paquets qui n'ont jamais été prévus pour fonctionner ensemble). Pour les fonctionnalités qui ne sont pas disponibles nativement en Julia, il est très facile d'accéder à du code écrit dans d'autres langages : C, Fortran, mais aussi Python, R ou MATLAB.
Cet atelier présentera les bases de la syntaxe de Julia et montrera son importance dans le cadre de projets de science des données. Notamment, il décrira brièvement DataFrames.jl, qui fournit une interface très similaire à Pandas en Python ou DataFrame en R, mais aussi Graphs.jl, une implémentation très performante d'algorithmes de graphe génériques, aussi simple à utiliser que NetworkX en Python mais bien plus rapide.
View details
No Results Found