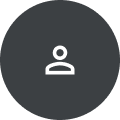
Pendo Abbo
Authored Publications
Sort By
Bridging the Gap: Unpacking the Hidden Challenges in Knowledge Distillation for Online Ranking Systems
Shuo Yang
Aniruddh Nath
Yang Liu
Li Wei
Shawn Andrews
Maciej Kula
Jarrod Kahn
Zhe Zhao
Lichan Hong
Preview abstract
Knowledge Distillation (KD) is a powerful approach for compressing large models into smaller, more efficient models, particularly beneficial for latency-sensitive applications like recommender systems. However, current KD research predominantly focuses on Computer Vision (CV) and NLP tasks, overlooking unique data characteristics and challenges inherent to recommender systems. This paper addresses these overlooked challenges, specifically: (1) mitigating data distribution shifts between teacher and student models, (2) efficiently identifying optimal teacher configurations within time and budgetary constraints, and (3) enabling computationally efficient and rapid sharing of teacher labels to support multiple students. We present a robust KD system developed and rigorously evaluated on multiple large-scale personalized video recommendation systems within Google. Our live experiment results demonstrate significant improvements in student model performance while ensuring the consistent and reliable generation of high-quality teacher labels from continuous data streams.
View details