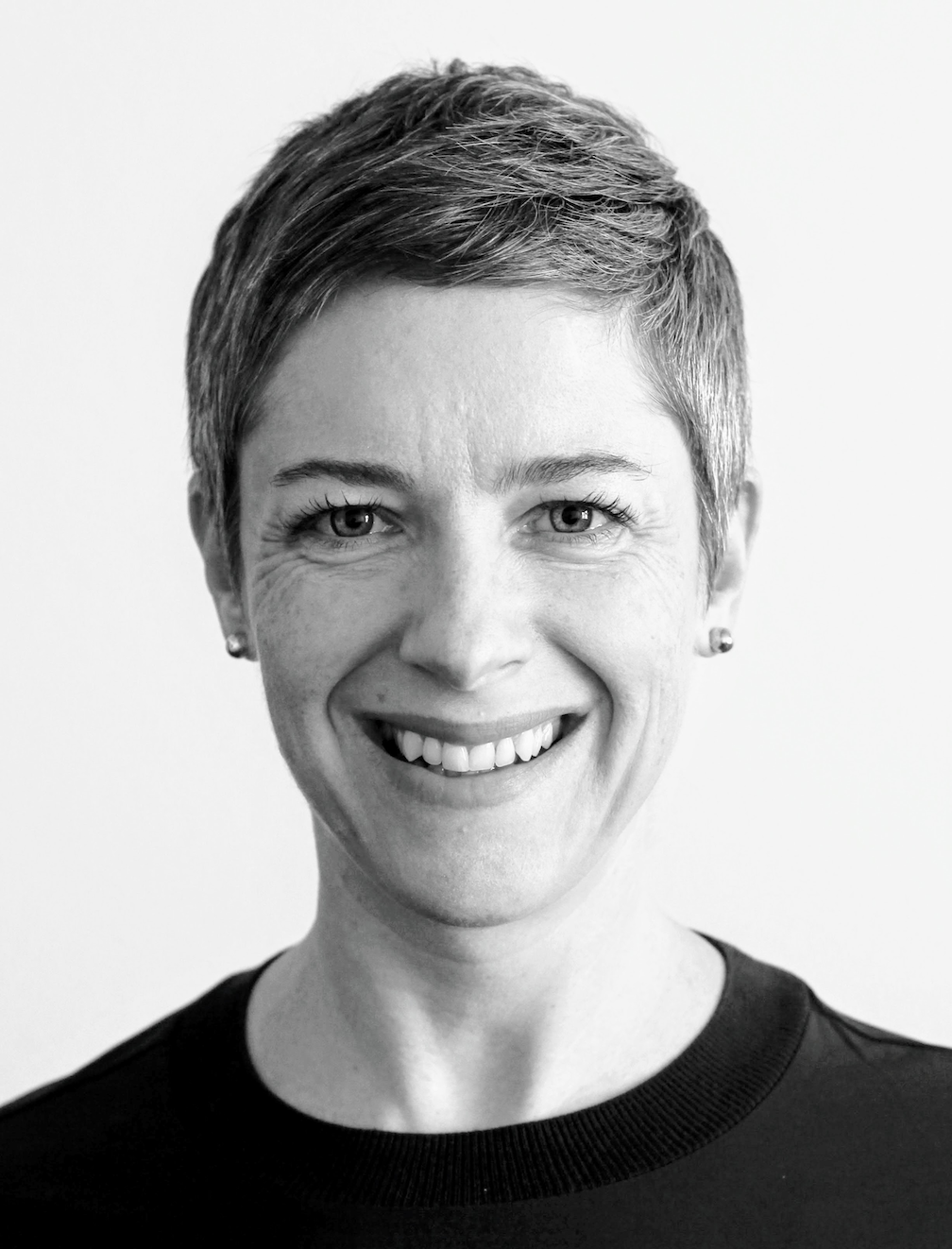
Caroline Pantofaru
Research Areas
Authored Publications
Sort By
FILM: Frame Interpolation for Large Motion
Fitsum Reda
Eric Tabellion
Proceedings of the European conference on computer vision (ECCV) (2022)
Preview abstract
We present a frame interpolation algorithm that synthesizes
an engaging slow-motion video from near-duplicate photos which often
exhibit large scene motion. Near-duplicates interpolation is an interesting
new application, but large motion poses challenges to existing methods.
To address this issue, we adapt a feature extractor that shares weights
across the scales, and present a “scale-agnostic” motion estimator. It
relies on the intuition that large motion at finer scales should be similar
to small motion at coarser scales, which boosts the number of available
pixels for large motion supervision. To inpaint wide disocclusions caused
by large motion and synthesize crisp frames, we propose to optimize
our network with the Gram matrix loss that measures the correlation
difference between features. To simplify the training process, we further
propose a unified single-network approach that removes the reliance on
additional optical-flow or depth network and is trainable from frame
triplets alone. Our approach outperforms state-of-the-art methods on
the Xiph large motion benchmark while performing favorably on Vimeo90K, Middlebury and UCF101. Source codes and pre-trained models are
available at https://film-net.github.io.
View details
Panoptic Neural Fields: A Semantic Object-Aware Neural Scene Representation
Kyle Genova
Xiaoqi Yin
Leonidas Guibas
Frank Dellaert
Conference on Computer Vision and Pattern Recognition (2022)
Preview abstract
We present Panoptic Neural Fields (PNF), an object-aware neural scene representation that decomposes a scene into a set of objects (things) and background (stuff). Each object is represented by an oriented 3D bounding box and a multi-layer perceptron (MLP) that takes position, direction, and time and outputs density and radiance. The background stuff is represented by a similar MLP that additionally outputs semantic labels. Each object MLPs are instance-specific and thus can be smaller and faster than previous object-aware approaches, while still leveraging category-specific priors incorporated via meta-learned initialization. Our model builds a panoptic radiance field representation of any scene from just color images. We use off-the-shelf algorithms to predict camera poses, object tracks, and 2D image semantic segmentations. Then we jointly optimize the MLP weights and bounding box parameters using analysis-by-synthesis with self-supervision from color images and pseudo-supervision from predicted semantic segmentations. During experiments with real-world dynamic scenes, we find that our model can be used effectively for several tasks like novel view synthesis, 2D panoptic segmentation, 3D scene editing, and multiview depth prediction.
View details
A Step Toward More Inclusive People Annotations for Fairness
Vittorio Ferrari
Proceedings of the AAAI/ACM Conference on AI, Ethics, and Society (2021)
Preview abstract
The Open Images Dataset contains approximately 9 million images and is a widely accepted dataset for computer vision research. As is common practice for large datasets, the annotations are not exhaustive, with bounding boxes and attribute labels for only a subset of the classes in each image. In this paper, we present a new set of annotations on a subset of the Open Images dataset called the ``MIAP (More Inclusive Annotations for People)'' subset, containing bounding boxes and attributes for all of the people visible in those images. The attributes and labeling methodology for the ``MIAP'' subset were designed to enable research into model fairness. In addition, we analyze the original annotation methodology for the person class and its subclasses, discussing the resulting patterns in order to inform future annotation efforts. By considering both the original and exhaustive annotation sets, researchers can also now study how systematic patterns in training annotations affect modeling.
View details
DOPS: Learning to Detect 3D Objects and Predict their 3D Shapes
Mahyar Najibi
Zhichao Lu
Vivek Mansing Rathod
Larry S. Davis
CVPR 2020
Preview abstract
We propose DOPS, a fast single-stage 3D object detection method for LIDAR data. Previous methods often make
domain-specific design decisions, for example projecting
points into a bird-eye view image in autonomous driving scenarios. In contrast, we propose a general-purpose
method that works on both indoor and outdoor scenes. The
core novelty of our method is a fast, single-pass architecture
that both detects objects in 3D and estimates their shapes.
3D bounding box parameters are estimated in one pass for
every point, aggregated through graph convolutions, and
fed into a branch of the network that predicts latent codes
representing the shape of each detected object. The latent shape space and shape decoder are learned on a synthetic dataset and then used as supervision for the end-toend training of the 3D object detection pipeline. Thus our
model is able to extract shapes without access to groundtruth shape information in the target dataset. During experiments, we find that our proposed method achieves stateof-the-art results by ∼5% on object detection in ScanNet
scenes, and it gets top results by 3.4% in the Waymo Open
Dataset, while reproducing the shapes of detected cars.
View details
Virtual Multi-view Fusion for 3D Semantic Segmentation
Xiaoqi(Michael) Yin
Brian Brewington
European Conference on Computer Vision (2020)
Preview abstract
Semantic segmentation of 3D meshes is an important problem for 3D scene understanding. In this paper we revisit the classic multiview representation of 3D meshes and study several techniques that make them effective for 3D semantic segmentation of meshes. Given a 3D mesh reconstructed from RGBD sensors, our method effectively chooses different virtual views of the 3D mesh and renders multiple 2D channels for training an effective 2D semantic segmentation model. Features from multiple per view predictions are finally fused on 3D mesh vertices to predict mesh semantic segmentation labels. Using the large scale indoor 3D semantic segmentation benchmark of ScanNet, we show that our virtual views enable more effective training of 2D semantic segmentation networks than previous multiview approaches. When the 2D per pixel predictions are aggregated on 3D surfaces, our virtual multiview fusion method is able to achieve significantly better 3D semantic segmentation results compared to all prior multiview approaches and competitive with recent 3D convolution approaches.
View details
AVA-ActiveSpeaker: An Audio-Visual Dataset for Active Speaker Detection
Ondrej Klejch
Radhika Marvin
Liat Kaver
Sharadh Ramaswamy
Arkadiusz Stopczynski
ICASSP, IEEE (2020)
Preview abstract
Active speaker detection is an important component in video analysis algorithms for applications such as speaker diarization, video re-targeting for meetings, speech enhancement, and human-robot interaction. The absence of a large, carefully labeled audio-visual active speaker dataset has limited evaluation in terms of data diversity, environments, and accuracy. In this paper, we present the AVA Active Speaker detection dataset (AVA-ActiveSpeaker) which has been publicly released to facilitate algorithm development and comparison. It contains temporally labeled face tracks in videos, where each face instance is labeled as speaking or not, and whether the speech is audible. The dataset contains about 3.65 million human labeled frames spanning 38.5 hours. We also introduce a state-of-the-art, jointly trained audio-visual model for real-time active speaker detection and compare several variants. The evaluation clearly demonstrates a significant gain due to audio-visual modeling and temporal integration over multiple frames.
View details
An LSTM Approach to Temporal 3D Object Detection in LiDAR Point Clouds
Rui Huang
Wanyue Zhang
ECCV (2020)
Preview abstract
Detecting objects in 3D LiDAR data is a core technology for autonomous driving and other robotics applications. Although LiDAR data is acquired over time, most of the 3D object detection algorithms propose object bounding boxes independently for each frame and neglect the useful information available in the temporal domain. To address this problem, in this paper we propose a sparse LSTM-based multi-frame 3d object detection algorithm. We use a U-Net style 3D sparse convolution network to extract features for each frame's LiDAR point-cloud. These features are fed to the LSTM module together with the hidden and memory features from last frame to predict the 3d objects in the current frame as well as hidden and memory features that are passed to the next frame. Experiments on the Waymo Open Dataset show that our algorithm outperforms the traditional frame by frame approach by 7.5% mAP@0.7 and other multi-frame approaches by 1.2% while using less memory and computation per frame. To the best of our knowledge, this is the first work to use an LSTM for 3D object detection in sparse point clouds.
View details
Pillar-based Object Detection for Autonomous Driving
Yue Wang
Justin Solomon
ECCV (2020)
Preview abstract
We present a simple and flexible object detection framework optimized for autonomous driving. Building on the observation that point clouds in this application are extremely sparse, we propose a practical pillar-based approach to fix the imbalance issue caused by anchors. In particular, our algorithm incorporates a cylindrical projection into multi-view feature learning, predicts bounding box parameters per pillar rather than per point or per anchor, and includes an aligned pillar-to-point projection module to improve the final prediction. Our anchor-free approach avoids hyperparameter search associated with past methods, simplifying 3D object detection while significantly improving upon state-of-the-art.
View details
Identifying interesting portions of videos
Preview
Arthur Wait
Krishna Bharat
Christian Frueh
Ryan Michael Hickman
Patent (2019)