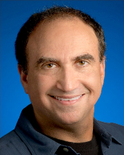
Yossi Matias
Yossi is the global exec lead of Google’s Health AI, driving AI research to help transform healthcare, and help make healthcare more accessible for everyone, with multiple breakthroughs including Med-PaLM. A leader of AI for Climate and Sustainability, he works on climate crisis mitigation (e.g., Greenlight) as well as adaptation - as the founding lead of Google’s Crisis Response initiative (SOS alerts, flood forecasting, wildfire detection). His work on Generative Ai includes efforts on Factuality (inc leading to Bard’s Double Check) and Efficiency (Speculative Decoding). Yossi pioneered Conversational AI innovations towards ambient intelligence to help transforming the phone experience (Google Duplex, Call Screen, Hold for Me) and help remove barriers of modality and languages (Live Caption, Live Relay, Euphonia, Read Aloud). He is a founding lead of Google’s AI for Social Good, Google for Startup Accelerator (with particular focus on AI & ML), Startups for Sustainable Development and Mind the Gap. He pioneered an initiative of bringing online hundreds of heritage collections (including the Dead Sea Scrolls), and helped establish Google’s Cultural Institute.
For over a decade Yossi was on Google’s Search leadership, driving strategic features and technologies (Autocomplete, Google Trends, Search Console, and Search experiences in weather, sports, dictionary and more). He is the founding managing director of Google’s center in Israel, (growing it to over 2500 on staff), supported Google’s growth (4X) in Bangalore, India, and oversees Google’s Expanding Research Center in Africa.
Yossi is on the Computer Science faculty at Tel Aviv University, and previously a Research Scientist at Bell Labs and visiting professor at Stanford. He’s published over 150 papers and is the inventor of over 70 patents. He pioneered some of the early technologies for internet privacy, contextual search, and the effective analysis of Big Data. He is a recipient of the Godel Prize, an ACM Fellow, and a recipient of the ACM Kanellakis Theory and Practice Award for seminal work on streaming algorithms, data sketches, and large-scale data analytics
Research Areas
-
Algorithms and Theory
-
Data Management
-
Data Mining and Modeling
-
Distributed Systems and Parallel Computing
-
Education Innovation
-
Health & Bioscience
-
Human-Computer Interaction and Visualization
-
Information Retrieval and the Web
-
Machine Intelligence
-
Natural Language Processing
-
Security, Privacy and Abuse Prevention
-
Speech Processing